Image Generation: Images and Human Judgements
- Type
-
ExperimentData
- Author
-
Mohammed Abdul Khaliq, Diego Frassinelli, Sabine Schulte im Walde
- Description
-
Nowadays tools for automatic image generation are accessible to laypeople as much as to experts. But do the generated images capture human mental representations? And which images are generated for abstract concepts and events that are not easily depictable, such as the concept patience and the event speak the truth, given that what we really see in the images depicting abstract knowledge are concrete objects?
We assess and compare four image generation models on how well they depict abstract vs. concrete event descriptions: DALL-E 2 (Ramesh et al., 2022), Stable Diffusion (Rombach et al., 2022), Stable Diffusion XL (Podell et al., 2023) and Midjourney (https://www.midjourney.com), as well as images retrieved by the search engine Bing (https://www.bing.com). The prompts for the models are represented by 40 phrase-level events consisting of a verb and a direct object noun, where we systematically vary the words’ degrees of abstractness by relying on the ratings in Brysbaert et al. (2014), cf. build a perspective vs. carry a box. We evaluate the generated images through human ratings (i) in a standard large-scale crowd-sourcing task, and (ii) in a two-step small-scale setup where we prime our participants on their expectations by asking them to first describe what they would expect to see in an image of a specific event, before asking them to judge the quality of the automatically generated images. Finally, (iii) we ask humans to judge about the metaphoricity (vs. literalness) of the underlying event targets, and to provide example sentences.
We provide the generated images as well as their human ratings across three annotation studies (see Download below).
- Reference
-
Mohammed Abdul Khaliq, Diego Frassinelli, Sabine Schulte im Walde (2024):
Comparison of Image Generation Models for Abstract and Concrete Event Descriptions
In: Proceedings of the 4th Workshop on Figurative Language Processing. Mexico City, Mexico. - Download
-
The annotation instructions and results can be found here.
For obtaining the generated images, please contact us.
SemRel
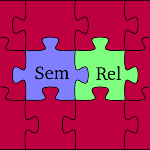
Research Group SemRel
- Write e-mail
- Research Group Sabine Schulte im Walde
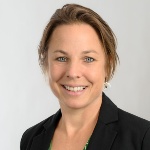
Sabine Schulte im Walde
Prof. Dr.Akademische Rätin (Associate Professor)