Improving Neural Political Statement Clasification
- Typ
-
Data and Models
- Autor
-
E. Dayanik, A. Blessing, N. Blokker, S. Haunss, J. Kuhn, G. Lapesa, S. Padó
- Referenz
-
(to be added when the paper is on the ACL anthology)
- Abstract
-
Many tasks in text-based computational social science (CSS) involve the classification of political statements into categories based on a domain-specific codebook. In order to be useful for CSS analysis, these categories must be fine-grained. The typically skewed distribution of fine-grained categories, however, results in a challenging classification problem on the NLP side. This paper proposes to make use of the hierarchical relations among categories typically present in such codebooks: e.g., markets and taxation are both subcategories of economy, while borders is a subcategory of security. We use these ontological relations as prior knowledge to establish additional constraints on the learned model, thus improving performance overall and in particular for infrequent categories. We evaluate several lightweight variants of this intuition by extending state-of-the-art transformer-based text classifiers on two datasets and multiple languages. We find the most consistent improvement for an approach based on regularization.
- Download
-
The data splits and models from the paper can be found at [URL to be added].
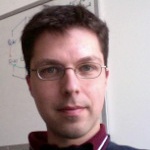
Sebastian Padó
Prof. Dr.Chair of Theoretical Computational Linguistics, Managing Director of the IMS