- Laufzeit
-
01/2021–12/2023
- PI
-
Roman Klinger
- Kurzbeschreibung
-
Emotion analysis and classification from text is typically phrased as an end-to-end machine learning task. In this project, we explore how to use knowledge from psychological theories to inform the task and improve predictive performance. We focus on appraisal theories.
- Geldgeber
-
Deutsche Forschungsgemeinschaft
- Langbeschreibung
-
Emotion analysis has typically been formulated as text classification task in which predefined emotion labels are assigned to textual units. The label set commonly follows the set of basic emotions as proposed by Ekman (Anger, Fear, Joy, Surprise, Sadness, Disgust) or Plutchik (adding Trust and Anticipation) or the valence-arousal-dominance model. This constitutes a gap between the state of research in psychology and computational linguistics, as the appraisal theories are widely accepted, but have not been used so far for emotion analysis in text.
With CEAT, we fill this gap and develop computational models of the cognitive appraisal of events and, to a lesser degree, of bodily symptoms and action tendencies. To represent the cognitive appraisal, we build on top of Smith/Ellsworth's (1985) work who show that the variables pleasantness, responsibility, certainty, attention, effort and situational control are sufficient to discriminate between a set of 15 emotions.
In this project, we create two approaches to assign these appraisal dimensions to textual event descriptions, firstly by building on top of semantic parsing and secondly in a deep learning setting. Based on these dimensions, we then predict the emotion associated with the textual fragment. This will lead to models that can automatically assign an emotion to an event description, even if no emotion words or self reports of feeling are available.
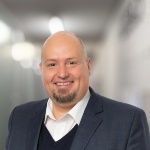
Roman Klinger
Prof. Dr.Außerplanmäßiger Professor