Willkommen am Lehrstuhl für Theoretische Computerlinguistik am IMS der Universität Stuttgart. Seit 2013 wird die Gruppe von Prof. Sebastian Padó geleitet.
Wir forschen in der Computerlinguistik, vor allem im Bereich der lexikalischen oder computergestützten Semantik, im Allgemeinen nach einem datengesteuerten Ansatz.
Hauptforschungsgebiete
Prof. Dr. Sebastian Padó, Chair
- Acquisition of lexical information: How can we automatically learn and extend lexicons from text that provide reliable information on various aspects of meaning and meaning variation?
- Semantic representation: What formalisms are available to represent such meaning information in a manner that is ideally both linguistically and cognitively adequate?
- Cross-lingual linguistic analysis: How can we use bilingual parallel and comparable corpora to learn more about linguistic structures in either language?
- From words to texts: What is the nature of the interaction between the meaning of lexical units, of phrases, sentences, and complete discourses?
- Applications of lexical semantics: How can all of the above contribute towards more intelligent and robust natural language processing applications that make a difference for the end user?
- Voreingenommenheit und Fairness in NLP: Wie können wir Voreingenommenheit in NLP-Modellen erkennen und verringern? Inwiefern beeinflussen demografische Variablen wie Geschlecht, Ethnizität oder sozioökonomischer Status die Ergebnisse von NLP-Systemen? Wie manifestieren sich Ungleichheiten in Primärdaten, und welchen Einfluss haben sie auf die Ergebnisse von NLP-Modellen?
- Erkennung und Handhabung schädlicher Sprache: Wie können NLP-Systeme schädliche oder beleidigende Sprache zuverlässig identifizieren? Welche Herausforderungen treten auf, wenn NLP-Systeme versuchen, subtile Formen von Schaden wie Mikroaggressionen oder implizite Voreingenommenheit zu erkennen?
- Rechnergestütztes Modellieren von sprachlicher Variabilität: Wie können wir sprachliche Variabilität modellieren, sodass die Bedeutung erhalten bleibt und Unterschiede im Ausdruck anerkannt werden? Wie entwerfen wir NLP-Systeme, die sich an die sprachlichen Präferenzen der Nutzer, einschließlich nicht-standardmäßiger Ausdrücke, anpassen?
- NLP für die rechnergestützte Sozialwissenschaft: Wie kann NLP zu interdisziplinärer Forschung beitragen, indem es neue Möglichkeiten bietet, sozialwissenschaftliche Daten zu modellieren und zu analysieren? Wie können NLP-Methoden verwendet werden, um große soziale Datensätze zu analysieren und Einblicke in das soziale Verhalten, Kommunikationsmuster oder öffentliche Meinungen zu gewinnen?
Projekte
Emotionsanalyse wurde bisher in der Regel als Textklassifikationsaufgabe formuliert, in der vordefininierte Klassen Textsegmenten zugewiesen wurden. Die Klassen entsprechen typischerweise den Basisemotion, wie Sie von Ekman (Wut, Angst, Freude, Überraschung, Traurigkeit, Ekel) oder Plutchik (zusätzlich Vertrauen und Antizipation) vorgeschlagen wurden. Eine weitere Alternative stellt das Valenz-Arousal-Dominanz-Modell als Referenzsystem dar. Diese Ansätze stellen allerdings einen Unterschied in dem Stand der Forschung zwischen Psychologie und komputationeller Linguistik dar, da in dem erstgenannten Feld die Appraisaltheorien akzeptiert sind, aber bisher nie fur Textanalyse genutzt wurden.
Diesen Unterschied im Forschungsstand der verschiedenen Disziplinen verkleinern wir mit dem Projekt CEAT. Wir erstellen komputationelle Modelle auf Basis des kognitiven Appraisals von Ereignissen und, zu einem geringeren Maße, auf Beschreibungen von körperlichen Reaktionen und der Motivationskomponente von Emotionen. Als Basis für die Modellierung des kognitiven Appraisals nutzen wir die Arbeiten von Smith/Ellsworth (1985), welche zeigten, dass die Variablen wie angenehm ein Ereignis ist, wie verantwortlich man sich fühlt, wie sicher man ist, wieviel Aufmerksamkeit man dem Ereignis entgegenbringt und wieviel situationelle Kontrolle man hat, ausreichend sind um zwischen 15 Emotionen zu diskriminieren.
In diesem Projekt erstellen wir zwei Modelle um diese Appraisaldimensionen textuellen Ereignisbeschreibungen zuzuweisen, zum einen auf Basis von semantischem Parsing, zum anderen auf Basis von tiefen neuronalen Netzen. Diese Dimensionen werden dann genutzt um die Emotion vorherzusagen, welche mit dem beschriebenen Ereignis wahrscheinlich verknüpft wird. Diese Modell werden erstmalig die Möglichkeit schaffen, Emotionen Ereignisbeschreibungen zuzuweisen, auch wenn Emotionsworte oder direkte Nennungen der Emotion nicht verfügbar sind.
Mitarbeiter: Roman Klinger (PI), Laura Oberländer, Enrica Troiano
Die Erforschung von Methoden zur automatischen Überprüfung von Fakten, also Computermodelle, welche korrekte Information von Fehlinformation oder Desinformation unterscheiden können, fokussiert weitestgehend auf die Nachrichtendomäne. So werden Nachrichten, auch solche, welche in sozialen Medien geteilt werden, auf ihren Wahrheitsgehalt überprüft. Solche Methoden sind bisher nicht für die biomedizinische Domäne entwickelt worden. Besondere Herausforderungen sind hier unter anderem die Reichhaltigkeit an existierenden (etablierten) Informationsquellen, die Komplexität der enthaltenen Information und der Unterschied der verwendeten Sprache von Experten und medizinischen Laien. In diesem Projekt entwickeln wir Informationsextraktionsysteme für Laien- und Expertensprache und Methoden um die extrahierten Informationen automatisch aufeinander abzubilden und in diesem gemeinsamen semantischen Raum Informationen automatisch abzugleichen, und schließlich auf ihren Wahrheitsgehalt unter Betrachtung von etablierten Quellen zu überprüfen. Das Projekt kombiniert somit Methoden des Transferlernens, der Informationsextraktion, und der Faktenüberprüfung für die biomedizinische Domäne insbesondere in sozialen Medien.
Mitarbeiter: Roman Klinger (Projektleitung), Amelie Wührl
Emotion analysis in natural language processings aims at associating text with emotions, for instance with anger, fear, joy, surprise, disgust or sadness. This task extends sentiment analysis, which adds further qualitative value in applications, for instance in social media analysis, in the analysis of fictional stories or news articles.Existing research has so far mainly focused on the association of text with specific emotion models from psychological research. The development of methods for detecting phrases in text which denote the emotion experiencer (the character or person who feels the emotion), the emotion theme (the cause of the development of an emotion) as well as the modifiers of an emotion (intensifiers and diminishers) has been neglected.In this project, we aim at filling this gap. We will develop manually annotated corpora from different domains (news, novels, social media) in German and English. Based on these resources, we develop models which are able to automatically recognize and extract such information. We work on different levels: Firstly, we connect words with emotions (with distributional and lexical methods), including grammatical variants. Then, secondly, we analyze these mentions in context with modifiers, the feeler and the theme (cause) of the emotion. Thirdly, we model these information in context, i.e., beyond seperated mentions. All methods will be analyzed regarding their domain and language independence.
Involved personnel: Roman Klinger (project lead), Laura Bostan, Evgeny Kim
This interdisciplinary collaboration project involving Computational Linguistics, Machine Learning and Political Science has the aim of developing new computational models and methods for analyzing argumentation in political discourse – specifically capturing the dynamics of discursive exchanges on controversial issues over time. The goal is to develop tools to support analysis of the possible impact of arguments advanced by different political actors.
Involved personnel: Jonas Kuhn, Sebastian Padó (project leads at IMS), Erenay Dayanik, André Blessing
In many kinds of prose texts, both literary or newswire texts, reportedspeech plays an important role as a source of information aboutcharacters, their attitudes, and their relationships. Going further,such information can aid in the analysis of patterns of behavior and theconstruction of social networks.While readers do not have any problem in assembling representations forcomplete situations from individual instances of reported speech, thisis still a challenging task for computers. Current state of the artmethods are generally organized as "pipelines" which start fromindividual instances of reported speech and proceed incrementally tomore global properties of the situation or characters. Since individualinstances of reported speech are often short and uninformative, apipeline procedure often causes prediction errors which cannot berectified in retrospect.In this project, we develop joint inference methods to model the variousaspects of reported speech (who is the speaker? the hearer? What is thecontent? What is the relationship between speaker and hearer?) togetherinstead of individually. The resulting joint model takes account of theinterdependencies between these aspects. Thus, information from thedifferent aspects can complement each other. The result of this part ofthe project is a solid starting place (in terms of natural languageprocessing methods) for the application of such methods for theautomatic analysis of reported speech in digital humanities and socialsciences.This algorithmic goal is complemented by a goal from corpus andcomputational linguistics, namely elucidating the relationship betweenreported speech and other aspects of semantic analysis. In particular,there appears to be a close relationship between reported speech and (asubset) of semantic roles. Yet, no comprehensive formal analysis hasbeen carried out so far. We will provide a linguistic characterizationof the relationship and exploit it algorithmically to further improvethe recognition of reported speech as discussed above. The results ofthis part of the project is the (at least partial) consolidation of twostrands of research that have largely been treated as independent sofar.
Involved personnel: Sebastian Padó (project lead), Sean Papay
Newspapers were the first big data for a mass audience. Their dramatic expansion over the long nineteenth century created a global culture of abundant information. Yet the significance of the newspaper has largely been defined in national terms in literary-historical scholarship of the period, and newspapers are predominantly collected, digitized, and accessed through nationally-focused institutions. "Oceanic Exchanges: Tracing Global Information Networks in Historical Newspaper Repositories, 1840-1914" (OcEx) brings together leading efforts in computational periodicals research to examine patterns of information flow across national and linguistic boundaries in nineteenth century newspapers and to link insights across large-scale corpora of digitized newspapers from national collections. For scholars of nineteenth century periodical culture and intellectual history, OcEx reframes how we understand the historical emergence of a globally-connected information network. It uncovers the ways that the international was refracted through the local as news, advice, vignettes, popular science, poetry, fiction, and more, all circulating around the globe and through multiple translations. By revealing the global networks through which texts and topics traveled in the period, OcEx promises to create an abundance of new evidence about how readers around the world perceived each other through the newspaper, evidence that will be of great interest to scholars in various fields. Computational linguistics and visualization provide a number of building blocks (recognizing translation, paraphrasing, text reuse, etc.) that can play enabling roles in scholarly investigations, with both historical and contemporary implications. At the same time, such methods raise fundamental questions regarding the validity and reliability of their results (such as the effects of noise in optical character recognition). Finally, by linking research across large-scale digital newspaper collections, OcEx will offer a model for national libraries and others developing large-scale data for digital scholarship. In tracing the ways texts, topics, and concepts crossed national and linguistic boundaries, Oceanic Exchanges seeks to break through the conceptual, institutional, and political barriers which have limited the promise of big data in the humanities: by bringing together historical newspaper experts from different countries and disciplines around common questions; by actively crossing the national boundaries that have previously separated digitized newspaper corpora, as well as those dividing public and private collections, through computational analysis; and by illustrating and making the global connectedness of nineteenth-century newspapers interactively explorable in ways hidden by typical organizations of digital cultural heritage along national lines.
Involved Personnell at IMS: Sebastian Padó, Martin Riedl
Website: http://oceanicexchanges.org/
CRETA is a BMBF-funded center for digital humanities whose goal is to collectively develop, test and use methods for reflected text analysis across text-oriented disciplines, connecting humanities subjects with computer science methods. The center will focus on methodological building blocks that are or can be used in more than one discipline and that will allow critically reflected insights into the topics under investigation. Our group's involvement is primarily in using language technology to further literary studies, notably by assigning emotions to characters in narrative text.
Involved personnel: Roman Klinger (project lead), Sebastian Padó (project lead), Evgeny Kim
More information: http://creta.uni-stuttgart.de
KABI is a project funded by the program “RiSC – Research Seed Capital” of the State Ministry of Baden-Württemberg for Sciences, Research and Arts, proposed by Roman Klinger. In the Life Sciences, most information is only available in free text in scientific publications. Automatic methods to extract such knowledge and to provide it in structured databases is challenged by a dilemma: Especially if potentially new information is detected in text, it is unclear if this information is actually correct or if it is wrongly extracted, for instance because the text is formulated in an uncommon way. In this project, methods will be developed which help to estimate the reliability of extracted information from biomedical publications.
Involved personnel: Roman Klinger (project lead), Camilo Thorne
More information
Derivational morphology is an important process of word formation. Work in computational linguistics has usually focused on the orthographic level, modeling derivation as a string transformation. The semantic level, where orthographic derivation patterns such as -er, -ung correspond to a variety of semantic shifts, has received less attention in the field.
The goal of this project is to model the semantics of derivational patterns using distributional methods. We will work in the recently developed framework of compositional distributional semantic models (CDSMs) which assumes that derivation is essentially a compositional process in which derivational patterns act as functors (represented as linear maps) that are applied to base terms (represented as vectors).
The goal of this project is to contribute to research on tensor-based compositional distributional seman- tic models by developing a syntax-semantics interface with three properties: (a) it will be dependency- based rather than based on constituents; (b) it will be incremental, that is, construct semantics in a left-to-right manner; (c) it will incorporate a notion of plausibility for (partial) analyses based on expecta- tions at the level of individual composition operations. The first property is important to develop syntax- semantics interfaces for languages with a more free word order. The second and third are well-known properties of human sentence processing.
There are two interleaved high-level goals for this project. The first is to set up, for the first time, a generic architecture and a comprehensive implementation for a multilingual textual inference platform and to make it available to the scientific and technological communities. The second goal of the project is to develop a new generation of inference-based industrial text exploration applications for customer interactions, which will enable businesses to better analyze and make sense of their diverse and often unpredicted client content. These goals will be achieved for three languages – English, German and Italian, and for three customer interaction channels – speech (transcriptions), email and social media. This is a EC STREP project undertaken in collaboration with Bar Ilan University, DFKI, FBK, and the companies AlmaWave, NICE, and OMQ.
The goal of the doctoral program is to extend semantic analysis to the discourse level and to approximate coherence-based interpretation through three mutually supporting research directions: (a) analysing semantic phenomena at the discourse level and representing them as “semantic graphs”; (b) using these graphs to improve semantic analysis; (c) evaluating (a) and (b) in NLP applications. The proposed PhD topics are integrated into and linked by the three directions. Further ties are ensured by the joint use of text collections (corpora).
Kooperationen
- Prof. Gemma Boleda, University Pompeu Fabra, Barcelona
- Prof. Hanno Ehrlicher, University of Tübingen
- Prof. Sebastian Haunss, University of Bremen
- Prof. Roman Klinger, University of Bamberg
- Prof. Alessandro Lenci, University of Pisa
- Prof. Jan Snajder, University of Zagreb
Abteilung Theoretische Computerlinguistik
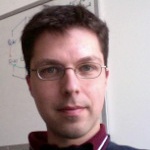
Sebastian Padó
Prof. Dr.Lehrstuhlinhaber Theoretische Computerlinguistik, Geschäftführender Direktor des IMS

Karin Leonte
Sekretariat für DP und TCL, studentische Angelegenheiten abteilungsübergreifend

Sabine Mohr
Dr.Sekretariat für GCL und CL, Projektverwaltung abteilungsübergreifend