Sommersemester 2024
Michael Franke:
The pragmatics of communicating causal information
Dienstag, 23. April um 17.30 Uhr in K2/17.12
Knowledge of causal processes is vital for all aspects of our lives. Yet while strong evidence for causal relations comes from interventionist experimentation, much of our causal knowledge is acquired not from individual experience or direct experimentation, but indirectly from cultural transmission via language. From this point of view, it is very curious that relatively little work in pragmatics has addressed the problem of communicating causal information. This talk therefore introduces a probabilistic model of communicating causal information, in which speakers choose utterances to inform listeners about causal facts, and in which listeners reason about alternative causal models based on the usual conversational assumptions of informative and relevant information exchange. We show that listeners can infer causal information reliably from expressions, like indicative conditionals, which arguably do not encode this information in their semantic meaning. We also address interesting puzzles in the association of cause and effect for certain kinds of correlational statements like “A is associated with B”.
Gastgeber: Daniel Hole & Judith Tonhauser
Alexander Pfaff
Montag, 6. Mai um 14.00 Uhr in FZI/V5.01
Gastgeber: Dominik Schlechtweg
Verna Dankers
Montag, 13. Mai um 14.00 Uhr in FZI/V5.01
Gastgeber: Prisca Piccirilli & Filip Miletić
Max van Duijn:
Language, Stories, and the Emergence of Socio-Cognitive Skills in Children and Large Language Models
Montag, 3. Juni um 14.00 Uhr in FZI/V5.01
Gastgeberin: Esra Dönmez
Evidence from various research traditions suggests strong, bi-directional links between language, narrative, and children’s socio-cognitive skills. For instance, performance on tasks involving Theory of Mind (ToM), i.e. the ability to attribute mental states to others and model the world from their perspective, has been shown to rely heavily on landmarks in linguistic and narrative development. Reversely, acquisition of certain lexical and syntactic competencies seems to boost children’s performance on ToM tasks, even when tested in non-linguistic ways. In this talk, I will discuss three recent studies from my lab contributing to this debate. We have created ChiSCor, a database of 700+ fantasy stories told by children aged 4-12, which we analyse using a combination of manual annotation, NLP techniques, and statistical modelling. The first study revolves around the representation of characters and their emotions, perceptions, and cognitive states, across different age groups. The second study models the occurrence of increasingly “deep” or mentally sophisticated characters as a function of context variables such as lexical and syntactic complexity. In the third study we used data and methods from the children’s story project to assess the potential capacity for ToM in Large Language Models (LLMs) of different sorts and sizes, such as LLaMA, Falcon, and GPT-4. I will conclude by reflecting on how recent advancements in NLP can inform long-standing debates on the relationship between language and socio-cognitive development, while at the same time these debates reflect back on how we should understand emerging cognitive capacities in artificially intelligent systems such as LLMs.
Lebenslauf: Max van Duijn is an assistant professor at Leiden University’s Institute of Advanced Computer Science (LIACS), where he co-founded the Creative Intelligence Lab (CIL). This lab brings together researchers from the cognitive and computer sciences who have a shared interest in the foundations of intelligence, both ‘in carbo’ and ‘in silico’, and who recognise creativity as a key factor in scientific innovation. Research in his group combines methods from cognitive science, linguistics, and AI to study social intelligence, in particular Theory of Mind. His work includes modelling these capacities in humans as well as in AI systems such as Large Language Models.
His NWO Veni project 'A Telling Story' (2020-2024) set out to collect a large number of stories told by children aged 4-12 and extract features relevant to ‘fictional minds’ using computational techniques. This fuelled new ways in which the development of (advanced) social intelligence can be modelled and understood across primary-school ages. In addition, he is part of the NWO Zwaartekracht 'Hybrid Intelligence' and the 'AI4Oversight' ICAI Lab. He is a lecturer in the Data Science & Artificial Intelligence BSc (DSAI) and Media Technology MSc programmes.
Max van Duijn’s work is published in English and Dutch, in scholarly as well as popularising venues. In 2023 he was elected as a member of De Jonge Academie, the early-career chapter of the Dutch Royal Academy of Sciences (KNAW), where he is co-chair of the Science & Society track and founder of the Generative AI in Research & Education theme group.
Wei Zhao
Montag, 10. Juni um 14.00 Uhr in FZI/V5.01
Gastgeber: Dominik Schlechtweg & Sabine Schulte im Walde
Wintersemester 2023 / 2024
Mark Dingemanse & Andreas Liesenfeld:
From text to talk: crossing human interaction and language technology
Montag, 6. November, um 14.00 Uhr in FZI/V5.01
Gastgeber: Sebastian Padó
As interactive language technologies increasingly become part of our everyday lives, insights from research on language and social interaction become more important to text-oriented fields like NLP and computational linguistics. We present recent work from our lab that aims to make these worlds meet. Point of departure is our call for a pivot from text to talk (Dingemanse & Liesenfeld 2022) in which we argue that conversational corpora harbour insights about turn-taking and timing, with implications for language typology, speech recognition and conversational interfaces. We discuss work on the typology of backchannels (continuers), humble words that are surprisingly important in streamlining human interactive language use yet that fall between the cracks of current conversational interfaces. We end by sketching some of the interactive affordances and constraints of large language models, and present some lessons learned while developing the first systematic survey of "open source" instruction-tuned LLMs, opening up ChatGPT.
Myrthe Reuver:
Democratically healthy news recommendation: aligning NLP with society, theory, and evaluation
Montag, 4. Dezember um 14.00 Uhr in FZI/V5.01 & Webex (hybrid)
Gastgeberin: Tanise Ceron
News recommender systems provide news article recommendations based on a user's interests and clicks. This personalization could harm democratic societies: citizens may be unaware of information beyond their own interests, leading to filter bubbles and a lack of shared public debate. A healthy collective information environment may require more diversity in recommendations, such as recommending different viewpoints on societal debates. Natural Language Processing could play a role in providing more diverse recommendations, but solving such a complex societal problem requires several key ingredients. These include interdisciplinary collaboration with experts on democracy, careful reflection on the suitability of existing NLP tasks and models, and data that is connected to relevant social science theory. Additionally, evaluation is essential: do we measure what we intend to measure, and is our NLP model actually improving recommendations in terms of our democratic values? I will discuss my PhD projects and findings - which include the lack of cross-topic robustness of stance detection models, and evaluation of models beyond predictive validity - and relate these to how we can tackle the problem of non-diverse news recommendation, and similar complex societal research questions.
Myrthe Reuver is a 4th year PhD candidate in the Computational Linguistics and Text Mining Lab (CLTL) at the Vrije Universiteit Amsterdam, with advisors prof.dr. Antske Fokkens (CLTL) and prof.dr. Suzan Verberne (Leiden University). Myrthe’s PhD is on analyzing diversity in news recommender systems, and her research has focussed on computational argumentation, precise evaluation, and interdisciplinary collaboration with social scientists and philosophers. Aside from her research papers, Myrthe has also applied NLP research during a summer internship at LinkedIn in Dublin, and has been featured in Dutch national newspapers about responsible use of AI.
Tilman Beck:
LLMs in Argument Mining
Montag, 11. Dezember um 14.15 Uhr in FZI/V5.01
Gastgeberin: Neele Falk
Detecting and classifying opinions is of interest for different use-cases, such as analyzing public opinion, social media analysis or political campaigning. In my talk I will present some of my works to improve language models to understand opinionated content. In particular, I will talk about the role of annotated data and how to integrate more context into the standard stance detection pipeline.
Sommersemester 2023
Nadine Bade, joint work with Emmanuel Chemla and Philippe Schlenker:
Word learning tasks as a window into the `triggering problem' for presuppositions
Dienstag, 25. April, um 15.45 Uhr in K2/17.25
Abstract: We show that native speakers spontaneously separate the complex meaning of a new word into a presuppositional component and an assertive component. These results argue for the existence of a productive triggering algorithm for presuppositions, one that is not based on alternative lexical items nor on contextual saliency. On a more methodological aspect, the proposed learning paradigm can be used to test further theories concerned with the interaction of lexical properties and conceptual biases.
Gastgeber: Daniel Hole & Judith Tonhauser
Michael Cysouw:
The daunting diversity of German diatheses
Dienstag, 23. Mai, um 15.45 Uhr in K2/17.12
Gastgeber: Daniel Hole & Judith Tonhauser
Isabelle Augenstein:
Beyond Fact Checking — Modelling Information Change in Scientific Communication
Montag, 26. Juni, um 14 Uhr in FZI V5.01/2
Gastgeber: Roman Klinger
Abstract: Most research on processing scholarly documents with natural language processing methods assumes that the information processed is trustworthy and factually correct. However, this is not always the case. There are two core challenges, which should be addressed: 1) ensuring that scientific publications are credible -- e.g. that claims are not made without supporting evidence, and that all relevant supporting evidence is provided; and 2) that scientific findings are not misrepresented, distorted or outright misreported when communicated by journalists or the general public. In this talk, I will present some first steps towards addressing these problems, discussing our research on exaggeration detection, scientific fact checking, and on modelling information change in scientific communication more broadly.
Bio: Isabelle Augenstein is a Professor at the University of Copenhagen, Department of Computer Science, where she heads the Copenhagen Natural Language Understanding research group as well as the Natural Language Processing section. Her main research interests are fact checking, low-resource learning, and explainability. Prior to starting a faculty position, she was a postdoctoral researcher at University College London, and before that a PhD student at the University of Sheffield. In October 2022, Isabelle Augenstein became Denmark’s youngest ever female full professor. She currently holds a prestigious ERC Starting Grant on 'Explainable and Robust Automatic Fact Checking', as well as the Danish equivalent of that, a DFF Sapere Aude Research Leader fellowship on 'Learning to Explain Attitudes on Social Media’. She is a member of the Young Royal Danish Academy of Sciences and Letters, and Vice President of SIGDAT, which organises the EMNLP conference series.
Simone Paolo Ponzetto:
Language Understanding for the Political Sciences
Tuesday, July 4th, 2 pm, Pfaffenwaldring 5c, V 5.03
Host: Agnieszka Falenska
Abstract. Over the past two decades, political scientists have increasingly adopted and developed natural language processing methods to use text as an additional source of data in their analyses. Over the last decade, the use of computational methods to analyse political texts has expanded significantly, leading to a substantial growth of the text-as-data community within political science. In this talk, I will present some of our recent contributions at the intersection of document understanding and automated analysis of political texts. I will also argue for the need for increasingly deeper levels of (linguistic) analysis in order to understand complex phenomena such as ideologies and political attitudes from texts.
Bio. Simone Paolo Ponzetto is professor of information systems at the university of Mannheim, where he leads the Natural Language Processing group. His main research interests lie in the areas of knowledge acquisition, text understanding, and the application of natural language processing methods for research in the digital humanities and computational social sciences.
Ines Rehbein
Towards modelling populist rhetoric in political text
Tuesday, July 11, Breitscheidstraße 2, room 2.41 (4th floor)
Host: Agnieszka Falenska
Abstract:
Populism is a complex concept that, due to its fuzziness, eludes strict definition. While most scholars agree that anti-elitism, people-centrism and a moralisation of the discourse are core components of populism, it is not yet clear how we can model it.
In the talk, we will discuss challenges for automatically detecting populist rhetoric in text and present first steps towards populism detection, focussing on the concept of "people-centrism". We then extend our approach to explore how politicians from different parties talk about members of "the people" and "the elite". Our work is relevant for the development of automatic measures of populism from text.
Bio: Ines Rehbein is a postdoctoral researcher in the Data and Web Science Group at the University of Mannheim, working with Prof. Dr. Simone Paolo Ponzetto and Prof. Dr. Heiner Stuckenschmidt. Her work is focussed on the adaptation and application of Natural Language Processing methods to research questions in the Computational Humanities and Computational Social Sciences. She has also worked in different areas of Computational Linguistics, including syntactic parsing, lexical semantics and discourse analysis.
Ines Rehbein
Towards modelling populist rhetoric in political text
Tuesday, July 11, Breitscheidstraße 2, room 2.41 (4th floor)
Host: Agnieszka Falenska
Abstract:
Populism is a complex concept that, due to its fuzziness, eludes strict definition. While most scholars agree that anti-elitism, people-centrism and a moralisation of the discourse are core components of populism, it is not yet clear how we can model it.
In the talk, we will discuss challenges for automatically detecting populist rhetoric in text and present first steps towards populism detection, focussing on the concept of "people-centrism". We then extend our approach to explore how politicians from different parties talk about members of "the people" and "the elite". Our work is relevant for the development of automatic measures of populism from text.
Bio: Ines Rehbein is a postdoctoral researcher in the Data and Web Science Group at the University of Mannheim, working with Prof. Dr. Simone Paolo Ponzetto and Prof. Dr. Heiner Stuckenschmidt. Her work is focussed on the adaptation and application of Natural Language Processing methods to research questions in the Computational Humanities and Computational Social Sciences. She has also worked in different areas of Computational Linguistics, including syntactic parsing, lexical semantics and discourse analysis.
Wintersemester 2022 / 2023
Yonatan Bisk:
Following Instructions and Asking Questions
Montag, 10. Oktober 2022, um 15 Uhr über WebEx:
https://unistuttgart.webex.com/meet/hsiu-yu.yang
Gastgeberin: Hsiu-yu Yang
As we move towards the creation of embodied agents that understand natural language, several new challenges and complexities arise for grounding (e.g. complex state spaces), planning (e.g. long horizons), and social interaction (e.g. asking for help or clarifications). In this talk, I'll discuss improvements to embodied instruction following within ALFRED and initial steps towards building agents that ask questions or model theory-of-mind.
Lifeng Han:
Cracking Multi-word Expressions in a nutshell: Neural MT walks on thin ice when facing MWEs
Dienstag, 11. Oktober 2022, um 11.30 Uhr am FZI in Raum V5.01
Gastgeber: Prisca Piccirilli & Sabine Schulte im Walde
Sigrid Beck:
Diachronic development of universal quantifiers: Middle English
Dienstag, 22. November 2022, um15.45 Uhr in K2/17.25
Gastgeber: Daniel Hole & Judith Tonhauser
Ekaterina Artemova:
Vote'n'Rank: Revision of Benchmarking with Social Choice Theory
Montag, 28. November 2022, um 14.00 Uhr am FZI in Raum V5.01 & WebEx (hybrid)
Gastgeber: Dominik Schlechtweg
The development of state-of-the-art systems in different applied areas of artificial intelligence (AI) is driven by benchmarks, which have played a crucial role in shaping the paradigm of evaluating generalisation capabilities from multiple perspectives. Although the paradigm is shifting towards more fine-grained evaluation across diverse complex tasks, the delicate question of how to aggregate the performances has received particular interest in the community. The benchmarks generally follow the unspoken utilitarian principles, where the systems are ranked based on their mean average score over task-specific metrics. Such aggregation procedure has been viewed as a sub-optimal evaluation protocol, which may have created the illusion of progress in the field. This paper proposes Vote'n'Rank, a framework for ranking systems in multi-task benchmarks under the principles of thesocial choice theory. We demonstrate that our approach can be efficiently utilised to draw new insights on benchmarking in several AI sub-fields and identify the best-performing systems in simulated practical scenarios that meet user needs. The Vote'n'Rank's procedures are empirically shown to be more robust than the mean average while being able to handle missing performance scores and determine conditions under which the system becomes the winner.
Lebenslauf: Ekaterina Artemova (LMU) holds a PostDoc position at MaiNLP Research Lab @CIS LMU. Prior to joining LMU, she was a research scientist at Huawei Noah’s Ark Lab, where she developed NLU systems, ranging from task-oriented dialog systems to information extraction pipelines. She also explores application of topological data analysis and creates challenge datasets for language model introspection.
Elisabeth Stark:
Non-maximal definites in Romance (focus on Francoprovencal)
Dienstag, 29. November 2022, um15.45 Uhr in K2/17.25
Gastgeber: Daniel Hole & Judith Tonhauser
Friederike Moltmann:
On the Syntax and Semantics of Special Quantifiers
Dienstag, 13. Dezember 2022, um15.45 Uhr in K2/17.25
Gastgeber: Daniel Hole & Judith Tonhauser
Quantifiers like something, everything, nothing, several things as well as pronouns like that and what (‘special quantifiers’ for short) have the exceptional ability of being able to replace nonreferential complements of various sorts without giving rise to substitution problems, as is illustrated for clausal complements of attitude verbs below:
(1)
a. John claims that he won.
c. John claims something / that / what Mary claims
b. ??? John claims a proposition / some entity / some content / some thing / it.
Special quantifiers are not only able to replace other nonreferential complements, such as predicative complements of copula verbs, complements of intensional transitives, direct quotes as complements of verbs of speaking, and complements of measure verbs. They are also able to replace definite and bare plural and mass DPs in extensional contexts.
(2) John ate two things, the beans and the rice / beans and rice.
Various philosophers have taken such phenomena to show that special quantifiers are substitutional quantifiers or genuine higher-order / plural / mass quantifiers. I will discuss a range of generalizations that pose serious difficulties for such views and that motivate the Nominalization Theory of such quantifiers and pronouns, namely on which they range over entities that would also be semantic values of suitable nominalizations (e.g. ‘claims’ in (1b). The Nominalization Theory faces the challenge, though, of providing a compositional semantic analysis. I will explore a novel syntactic and semantic analysis of special quantifiers within the Nominalization Theory on which –thing as a light noun (in Kayne’s sense) plays a central role.
Fabienne Martin
Scaling agents via Dimensions
Tuesday, Januar 10, at 15.45am in K2
Hosts: Daniel Hole, Judith Tonhauser
Susann Fischer (University of Hamburg)
The Person-Case constraint reloaded: Data from French
Wednesday, Januar 11, at 15.45am in K2 and online at:
https://unistuttgart.webex.com/unistuttgart/j.php?MTD=ma78742905d8f44230a23ae46b4974cb5 er beitreten
Meeting code: 2732 570 9494
Meeting password: 3CFtQMKNn36
Host: Achim Stein
Aaron Hirsch
"Only as a form-meaning mismatch"
Tuesday, Januar 10, at 15.45am in K2
Hosts: Daniel Hole, Judith Tonhauser
Guglielmo Inglese (Univ. Torino) & Anne Wolfsgruber (HU Berlin)
Intransitives and reflexive marking in Anglo-Norman: the role of animacy, genre and language contact
Wednesday, February 8, at 15.45 online at:
https://unistuttgart.webex.com/unistuttgart/j.php?MTID=mc9538a4b2968263a9972282f64c28ec0
Meeting code: 2731 193 8983
Meeting password: UHtF6ev73Fe
Abstract:
The Latin reflexive pronoun se famously developed into a middle marker in Romance languages, coming to cover a wide range of new functions, including valency-reducing operations such as anticausativization. Moreover, in the course of history, reflexive marking also became obligatory with a number of intransitive verbs, e.g. French s’évanouir ‘faint’. While the rise of new valency-related functions has been the object of detailed studies, the obligatorification of reflexive marking remains largely unexplored. By focusing on the Anglo-Norman variety of Old French, we first investigate possible motivations behind reflexive marking with intransitive verbs, including verb semantics and prefixation. We also look into the role of animacy, textual genre and language contact in the generalization of reflexive marking. We then test the hypothesis that intransitive reflexive marking started out as optional (or pleonastic), e.g. (se) gesir ‘lie down’, and that only some pleonastic verbs became reflexive-only verbs over time. Our results show that, while obligatorification may have been one decisive factor, the rise of reflexive-only verbs is a more complex phenomenon.
Host: Achim Stein
David Jurgens (U.Michigan)
Title: Beyond the Individual: Modeling Interpersonal Relationships in NLP Studies of Communication
When/Where: Monday 27 February, at 14.00, online FZI, Room V5.01 & WebEx (hybrid): unistuttgart.webex.com/meet/gabriella.lapesa
Abstract: NLP studies of communication often focus on the individual: What we say, when we say it, and how we say it. Work on user prediction has shown that aspects of the individual (e.g., gender, age) are revealed in our language. Yet, the larger social context beyond the individual also plays an important role in our communication. In this talk, I will describe three studies from my group that model interpersonal relationships as a part of the social context in order to understand communication practices. In the first part of the talk, I will introduce a large-scale study of over 9M self-declared relationships in social media and show how relationships influence the what and when in our communications. Further, I will show how we can use these observed communications in social media to infer the likely type of relationship between individuals and, in turn, how knowledge of relationships is useful for predicting behavior like content resharing. In the second part, I will discuss recent work showing how we can directly incorporate social relationships in NLP models to change a message’s interpretation based on the social context in which it is communicated. Last, in the third part, I will discuss ongoing work at modeling empathy in interpersonal communication using appraisal theory. In this approach, we measure empathy by explicitly modeling the listener’s theory of mind based on how speaker and listener align in their communication.
The speaker: David is an assistant professor in the School of Information at the University of Michigan. He previously obtained his PhD at the University of California and was a postdoctoral researcher in the Department of Computer Science at Stanford University. His research is known for its interdisciplinary character and combines NLP with network analysis, computational social science, data science and psychology. This way, he tries to gain a 'social understanding' of both the content and the people involved in communication.
Hosts: Sofie Labat & Gabriella Lapesa. To arrange a meeting with a speaker and/or participate in the dinner after the talk, contact gabriella.lapesa@ims.uni-stuttgart.de
Jackie Chi Kit Cheung:
What Can Summarization Do for NLP? Problem Settings, Task Definitions, and Solutions
Dienstag, 28. Februar, um 10 Uhr am FZI in Raum V5.01 & WebEx (hybrid): https://unistuttgart.webex.com/meet/sabine.schulte-im-walde
ChatGPT and other human-facing generative language models have become a hot topic in the NLP community and in popular society at large. These models represent a success for the field, but also a source of angst because of their potential for disruption. For example, they are known to generate inaccurate or biased text, and may impact the functioning of our research communities and societies. I propose text summarization as a good focusing use case that can help NLP researchers discuss and anticipate such issues, as text summarization is inherently geared towards human consumption and requires semantic processing. I will discuss illustrative studies from my lab on factuality and hallucination, on proposing new task definitions to expand the scope of NLP applications, and on the solutions that we have devised for several of these issues. Just as machine translation has driven many advances in the first decades of NLP (e.g., alignment algorithms, language models, evaluation practices), summarization has the potential to do so for the next decades.
Jackie Chi Kit Cheung is an associate professor at McGill University's School of Computer Science, where he co-directs the Reasoning and Learning Lab. He is a Canada CIFAR AI Chair and an Associate Scientific Co-Director at the Mila Quebec AI Institute. His research focuses on topics in natural language generation such as automatic summarization, and on integrating diverse knowledge sources into NLP systems for pragmatic and common-sense reasoning. He also works on applications of NLP to domains such as education, health, and language revitalization. He is a consulting researcher at Microsoft Research Montreal.
Gastgeber: Filip Miletic & Sabine Schulte im Walde
Sommersemester 2022
Eric Wallace:
Interpreting Predictions of NLP Models
Freitag, 29. April 2022, um 17.30 Uhr, über WebEx:
https://unistuttgart.webex.com/meet/agnieszka.falenska
Gastgeberin: Agnieszka Faleńska
Today's large pre-trained NLP models are empirically accurate, but they also systematically fail in counterintuitive ways and are opaque in their decision-making process. This talk will first provide an overview of pre-trained NLP models. We will then dive into existing work on methods to explain the predictions of NLP models, including saliency maps, input perturbations (e.g., LIME, input reduction), adversarial attacks, and influence functions. I will conclude with a high-level discussion of open problems in the field, e.g., evaluating, extending, and improving interpretation methods.
Eric Wallace (https://www.ericswallace.com/) is a third-year PhD student at UC Berkeley working on Machine Learning and Natural Language Processing. He is advised by Dan Klein and Dawn Song, and has affiliations with BAIR, Berkeley NLP, and Berkeley Security. His current research interests are centered around security and privacy, large language models, and robustness and generalization.
Elin McCready:
Dogwhistles and When to Abondon Them
Dienstag, 17. Mai, um 17.30 Uhr, in K2/17.12
Gastgeber: Daniel Hole & Judith Tonhauser
Dogwhistles are coded signals used to disguise one's social persona from some listeners while revealing it to others, usually those who are sympathetic with it. This talk sketches a theory of dogwhistles set in a game-theoretic context and considers two aspects of dogwhistle interpretation and use: how searching for them can lead to hypervigilance and consequent disruptions in social cohesion, and in what kinds of circumstances speakers choose to abandon dogwhistling for direct signaling of their personas, arguing that both these phenomena are intertwined.
Gabriella Vigliocco (joint work with Viktor Kewenig and Jeremy Skipper):
"Real-word processing of abstract and concrete concepts"
A number of studies have established processing differences between concrete and abstract concepts suggesting that at least partially segregable networks in the brain underscore their representation, with concrete concepts engaging more sensory-motor networks and abstract concepts engaging more the language and emotion networks. These results, however, have been obtained in experiments that do not resemble the conditions in which concepts are normally processed in the real-world because, in previous studies, concepts are presented as single words and because participants are usually asked to carry out artificial tasks (e.g., lexical decision). In this talk, I will present a study we carried out to investigate processing of concrete and abstract concepts as it occurs in real-world conditions. We took advantage of an existing corpus of Neuroimaging data (https://www.naturalistic-neuroimaging-database.org/) to establish: (1) the patterns of activation occurring during naturalistic processing (movie watching); (2) how the pattern of activation changes depending upon the visual context in which the words are embedded at the moment of processing.
Alessandro Lenci (joint work with Ludovica Cerini):
"Picturing abstract words"
Abstract words too are grounded in sensorimotor experiences. In fact, images convey abstract meanings associated with the entities they represent. For instance, the image of a book can evoke concepts like knowledge, wisdom, etc. In this talk, I will present ongoing work aiming at investigating the relationship between images and abstract words. We have been collecting norming data about word-word and word-picture production data, to identify the association strength between images and abstract lexical items. The goal of this research is study to what extent the abstract concepts triggered by images are mediated by linguistic associations, and to investigate the multimodal factors determining the effectiveness of a given image in triggering a given abstract concept.
Freitag, 20. Mai 2022, um 16 Uhr, hybrid: FZI/V5.01 & WebEx:
https://unistuttgart.webex.com/meet/sabine.schulte-im-walde
Gastgeber: Diego Frassinelli & Sabine Schulte im Walde
Florian Schäfer:
Transitive Causitives
Dienstag, 21. Juni, um 17.30 Uhr, in K2/17.12
Gastgeber: Daniel Hole & Judith Tonhauser
Patrick Helling:
Research Done Meaningfully: core aspects of Research Data Management from a pragmatic perspective
Donnerstag, 23. Juni 2022, um 9.45 Uhr, über WebEx:
https://unistuttgart.webex.com/meet/kerstin.jung
Gastgeberin: Kerstin Jung
Research data is a key element of ongoing scientific progress. Making research data findable, accessible, interoperable, and reusable in the sense of the FAIR Principles is a substantial aspect of good research practice. In this talk I will give a brief introduction in the core elements of research data management following the research data life cycle. Then, I will focus on the strategies and infrastructures for publishing research data and outcomes of research as well as on legal obstacles one can run into while doing so.
Bio:
Patrick Helling is a data manager at the Data Center for the Humanities (DCH) at the Faculty of Arts and Humanities at the University of Cologne. He supports humanities scholars in questions on research data management (RDM) and he provides domain specific RDM services. In addition, he is part of the data management team of the DFG priority program 2207 “Computational Literary Studies”. As data steward of the Verband “Digital Humanities im deutschsprachigen Raum” e.V. he develops a research data management strategy for the scholarly outcomes of the DHd-Community. He is PhD-candidate at the University of Cologne. His doctoral research concerns the creation of a formal description model for domain specific research data management requirements and services for the humanities.
Andreas Vlachos
Fact-checking as a conversation.
Abstract:
Misinformation is considered one of the major challenges of our times resulting in numerous efforts against it. Fact-checking, the task of assessing whether a claim is true or false, is considered a key weapon in reducing its impact. In the first part of this talk I will present our recent and ongoing work on automating this task using natural language processing, moving beyond simply classifying claims as true or false in the following aspects: returning evidence for the predictions, factually correcting the claims and adversarial evaluation. In the second part of this talk, I will present an alternative approach to combatting misinformation via dialogue agents, and present results on how internet users engage in constructive disagreements and problem-solving deliberation.
Webex link: https://unistuttgart.webex.com/meet/roman.klinger
Host: Amelie Wührl
Wintersemester 2021
Janosch Haber (Queen Mary University London):
Polysemy Patterns in Human Judgements and Contextualised Language Models
Montag, 25. Oktober 2021, 14 Uhr, hybrid: FZI, V5.01 / WebEx:
https://unistuttgart.webex.com/meet/sabine.schulte-im-walde
Gastgeber: Dominik Schlechtweg, Sabine Schulte im Walde
Zusammenfassung: How does our brain connect words to meaning? And how is word meaning represented in computational language models? Lexical ambiguity - the fact that some words can have multiple interpretations - has always been a major challenge in finding satisfactory answers to either of these questions. Addressing homonymy - where a word can have two or more completely different, unrelated interpretations ("The match ended without a winner" vs "The match burned his fingers") - recently has been one of the reasons for developing contextualised language models to represent words not as static encodings but relative to their individual use in context. Polysemy on the other hand - words with different distinct but related senses ("The newspaper fired its editor in chief" vs "The newspaper fell of the kitchen table") - has received a little less attention.
In my research, I show that polysemy is a complex, heterogenous phenomenon, with polysemic targets exhibiting effects ranging from (near) identity of meaning to full homonymy. Observing measurable distances between word sense interpretations in a novel, human-annotated dataset provides new evidence against a range of traditional models suggesting a fully under-specified representation of polysemic sense in the mental lexicon, and instead supports more recent models proposing a structured representation based on word sense similarity clustering. Besides informing (psycho-)linguistics research, the collected data also can be used as a new benchmark in evaluating the context sensitivity of computational language models, and indicates that off-the-shelf, contextualised language models like BERT already seem to capture a decent amount of polysemic sense.
Bio: Janosch Haber is a PhD student at Queen Mary University of London, working on ambiguity and under-specification in natural language. His current focus is on the representation of polysemous words in the mental lexicon and contextualised language models. He previously worked on establishing a large-scale dataset of visually-grounded, collaborative dialogue for the development of multi-modal language models. Field: Natural Language Processing, Computational Linguistics
Kajsa Djärv (Universität Konstanz):
Embedded main clause phenomena: new perspectives on embedded illocutionary acts
Dienstag, 2. November 2021, 15.45 Uhr, über WebEx:
https://unistuttgart.webex.com/meet/judith.tonhauser
Gastgeber: Daniel Hole, Judith Tonhauser
In this talk, I examine the claim that embedded verb second (EV2), a well-studied ‘main clause phenomenon’, is associated with the illocutionary force of assertion. I argue that the illocutionary force of EV2-clauses is best captured in terms of the statement that they share the conventional discourse effects typically associated with main clause declaratives; in the sense of Farkas and Roelofsen (2017). I show that this proposal nicely captures the core discourse effects associated with EV2, while still allowing for a wide range of attested uses (based on naturally occuring data from Swedish), including cases where the embedded clause is not asserted, but functions as a type of biased question (a type of use which is not readily captured by accounts that link the availability of EV2 to an assertive operator or feature, such as Krifka 2014, a.o). The current perspective also provides us with a nice way of understanding the role of the matrix predicate and the licensing restrictions on EV2. I also discuss consequences of the current account and the data discussed here, for theories of clausal complementation and theoretical modelling in inquisitive semantics.
Nikolay Arefyev (Lomonosov Moscow State University):
Fine-tuning of cross-lingual language models for lexical semantic change detection
Montag, 6. Dezember 2021, 14 Uhr, online über Webex
Gastgeber: Dominik Schlechtweg, Sabine Schulte im Walde
Contextualized word embeddings from out-of-the-box neural language and masked language models are known to be bad representations of word meaning due to large orthographic / grammatical bias. Fine-tuning such models on some labeled datasets for the final task is the standard way to achieve good performance, however, it is not obvious how to fine-tune them directly for the lexical semantic change detection (LSCD) task, where examples are single words and targets are some scores corresponding the change in their meaning between two time periods.
In this talk I will present our experiments on fine-tuning models for the Word-in-Context and the Word Sense Disambiguation tasks and applying them for solving the LSCD task. These models achieved two best results in the recent RuShiftEval-2021 shared task on lexical semantic change detection for the Russian language. We have investigated how the choice of the architecture, the training procedure and data affects the final results. Surprisingly, even models fine-tuned on English data only achieve SOTA performance due to zero-shot cross-lingual abilities of the underlying cross-lingual masked language model. We have also made a detailed error analysis for the best performing model and found some types of senses that are hard to distinguish for our model and likely other language and mask language models as well.
Stefanie Dipper (Ruhr-Universität Bochum):
Annotation Schemes for Analyzing Metaphors
Montag, 20. Dezember 2021, 14 Uhr, hybrid: FZI, V5.01 / WebEx:
https://unistuttgart.webex.com/meet/sabine.schulte-im-walde
Gastgeber: Prisca Piccirilli, Jonas Kuhn, Sabine Schulte im Walde
In this talk, I would like to present ongoing joint work on analyzing metaphor, mainly in religious language. Metaphor, defined as a "mapping across two conceptual domains" (Steen, 2007) is an ubiquitous phenomenon in language. It fulfills a special role in religious language in that it enables us to express ideas about an abstract entity by referring to a well-known concrete entity and, in this way, to make statements about the transcendent.
In the talk, I address different annotation schemes that have been proposed for annotating metaphorical expressions, with a focus on our ongoing work on annotating deliberate metaphors following the Five Step Method proposed by Steen (2007) and discuss the analysis of selected complex cases. In addition, I report on a pilot study in which we are applying Shutova et al's (2013) approach to German-language religious texts and present preliminary results from this study.
References:
Gerard J. Steen. 2007. "Finding Metaphor in Discourse: Pragglejaz and Beyond." Cultura, Lenguaje y Representación, 5:9–25.
Ekaterian Shutova, Simone Teufel and Anna Korhonen. 2013. "Statistical Metaphor Processing." Computational Linguistics 39 (2): 301-353.
Anne Lauscher (Bocconi University)
Improving dialog system performance and fairness for ensuring effective, efficient, and just communication
Montag, 17. Januar 2022, 14 Uhr, online über WebEx:
https://unistuttgart.webex.com/meet/gabriella.lapesa
Gastgeberinnen: Neele Falk, Gabriella Lapesa
Dialog systems are a key component of envisioned future communication processes.However, they still exhibit potential for performance improvements in various tasks, and little is known about unfair stereotypical biases potentially encoded in the models, which may jeopardize fair communication. In this talk, I will discuss our latest efforts to address both issues.
(1) While self-supervised dialog-specific pretraining on large conversational datasets yields substantial gains over traditional language modeling for pretraining task-oriented dialog systems, these approaches exploit general dialogic corpora (e.g., Reddit) and thus presumably fail to embed domain-specific knowledge useful for concrete downstream domains reliably. To address this issue, we propose DS-TOD, a novel domain specialization framework. Our experiments with two prominent TOD tasks – dialog state tracking and response retrieval – demonstrate the effectiveness of our specialization approach. Moreover, we show that light-weight adapter-based specialization performs comparably to full fine-tuning in single-domain setups and is particularly suitable for multi-domain specialization.
(2) The landscape of bias measurements and mitigation resources and methods for conversational language models is still very scarce. We present RedditBias, the first conversational data set grounded in the actual human conversations from Reddit, allowing for bias measurement and mitigation across four important bias dimensions: gender, race, religion, and queerness. Further, we develop an evaluation framework that simultaneously a) measures bias on the developed RedditBias resource and b) evaluates model capability in dialog tasks after model debiasing. We use the evaluation framework to benchmark the widely used conversational DialoGPT model along with the adaptations of four debiasing methods.
Speaker:
Anne Lauscher is a postdoctoral researcher in the Natural Language Processing group at Bocconi University in Milan, Italy, where she is working on introducing demographic factors into language processing systems with the aim of improving algorithmic performance and system fairness. Besides fairness in dialog systems and computational argumentation, Anne has also worked on multilingual language transfer (e.g., https://aclanthology.org/2020.emnlp-main.363/ ).
If you want to have a personal meeting with Anne, drop a mail to gabriella.lapesa@ims.uni-stuttgart.de
Craige Roberts (OSU und Barnard College):
Imperatives in a Dynamic Pragmatics
Dienstag, 1. Februar 2022, 15.45 Uhr, über WebEx:
https://unistuttgart.webex.com/meet/judith.tonhauser
Gastgeber: Daniel Hole, Judith Tonhauser
Abstract:
I offer a semantics and pragmatics for imperatives, developed in the framework of a dynamic pragmatics in the vein of Portner (2004, 2018, 2018b) and Roberts (1996/2012, 2012b, 2017, 2018). The denotation of an imperative is a property indexically directed to the addressee (as in Portner 2004, 2007, 2011; and Starr 2020), but it is modal (as in Kaufmann 2006 (as Schwager), 2012). However, the modal is futurate, and its deontic force derives from the pragmatics, much as in Portner, arising from the interaction between semantic content and the canonical use of imperative clauses: to propose an update to the addressee's goals, plans and priorities (rather than to the ToDo lists of Portner).
The resulting account has the virtues of the best previous accounts (in particular those of Portner; Kaufmann; and Charlow 2011, 2014), while avoiding empirical problems that arise in those accounts and others in the literature.
References:
Charlow, Nathan (2011) Practical language: Its meaning and use. Ph.D.Thesis, University of Michigan.
Charlow, Nate (2014) Logic and semantics for imperatives. Journal of Philosophical Logic 43:617-664.
Kaufmann, Magdalena (2006) (as Schwager) Interpreting imperatives. Ph.D. Dissertation, University of Frankfurt.
Kaufmann, Magdalena (2012) Interpreting Imperatives. Springer, Studies in Linguistics and Philosophy 88. Revised version of M. Schwager (2006) Ph.D. thesis, University of Frankfurt.
Kaufmann, Magdalena (2021) Imperatives. In Daniel Gutzmann, Lisa Matthewson, Cecile Meier, Hotze Rullmann & Thomas Ede Zimmermann (eds.) The Wiley Blackwell Companion to Semantics. John Wiley & Sons, Malden, MA. DOI: 10.1002/9781118788516.sem067.
Portner, Paul (2004) The semantics of imperatives within a theory of clause types. In K.Watanabe & R. Young (eds.) Proceedings of SALT 14. CLC Publications.
Portner, Paul (2007) Imperatives and modals. Natural Language Semantics 15:351-383.
Portner, Paul (2011) Permission and choice. In G. Grewendorf & T.E. Zimmermann (eds) Discourse and Grammar: From sentence types to lexical categories. Studies in Generative Grammar. Mouton de Gruyter, Berlin.
Portner, Paul (2017) Imperatives. In Maria Aloní & Paul Dekker (eds.) The Cambridge Handbook of Formal Semantics. Cambridge University Press., https://doi-org.proxy.ohiolink.edu:9100/10.1017/CBO9781139236157
Portner, Paul (2018) Mood. Oxford University Press.
Portner, Paul (2018b) Commitment to priorities. In Daniel Fogal, Daniel W. Harris & Matt Moss (eds.) New Work on Speech Acts. Oxford University Press, pp.297-316.
Roberts, Craige (1996/2012) Information Structure: Toward an integrated theory of formal pragmatics. Published, with a new afterword, in 2012 in Semantics and Pragmatics 5.7.
Rotem Dror (University of Pennsylvania)
Statistical Significance Testing for Natural Language Processing
Montag, 7. Februar 2022, 14.45 Uhr, online über WebEx:
https://unistuttgart.webex.com/meet/oezlem.cetinoglu
Gastgeberin: Özlem Cetinoglu
Abstract:
Data-driven experimental analysis has become the main evaluation tool of Natural Language Processing (NLP) algorithms. In fact, in the last decade, it has become rare to see an NLP paper, particularly one that proposes a new algorithm, that does not include extensive experimental analysis, and the number of involved tasks, datasets, domains, and languages is constantly growing. This emphasis on empirical results highlights the role of statistical significance testing in NLP research: If we, as a community, rely on empirical evaluation to validate our hypotheses and reveal the correct language processing mechanisms, we better be sure that our results are not coincidental.
In this talk, I will go through the main chapters of the book in the title (https://www.morganclaypool.com/doi/abs/10.2200/S00994ED1V01Y202002HLT045) and answer the following questions: How to choose a valid statistical test for your experiments? How to perform statistical analysis when experimenting with multiple datasets? How to compare deep neural models in a statistically valid manner?
Bio:
Rotem Dror is a Postdoctoral Researcher at the Cognitive Computation Group at the Department of Computer and Information Science, University of Pennsylvania, working with Prof. Dan Roth. Rotem has completed her Ph.D. in the Natural Language Processing Group, supervised by Prof. Roi Reichart, at the Faculty of Industrial Engineering and Management at the Technion - Israel Institute of Technology. Rotem is a recipient of the Google Ph.D. Fellowship 2018 and the Eric and Wendy Schmidt Postdoctoral Award for Women in Mathematical and Computing Sciences.
Malte Zimmermann (Universität Potsdam) & Reginald Akuoko Duah (University of Ghana, Legon & Humboldt Universität zu Berlin):
Situation anaphoricity in Hausa and Akan: A uniform account
Dienstag, 8. Februar 2022, 15.45 Uhr, über WebEx:
https://unistuttgart.webex.com/meet/judith.tonhauser
Gastgeber: Daniel Hole, Judith Tonhauser
Abstract:
The presentation focuses on two formal strategies of coding situation anaphoricity in two un-related tenseless languages, namely Hausa (Chadic) and Akan (Kwa). The two formal markers are shown to involve the same underlying situation semantics (including salient context situations, Austinian topic situations, and situation extension), thereby accounting for their parallel distribution in the two languages. Hausa and Akan differ, though, in that Akan uses grammatical tone to disambiguate between situation-related and tense-related anaphoricity.
Sommersemester 2021
Sameer Singh (University of California, Irvine):
Evaluating and Testing Natural Language Processing Models
Montag, 19. April 2021, 17 Uhr, cancelled über WebEx:
https://unistuttgart.webex.com/unistuttgart-en/j.php?MTID=m4ecc38dc6efbde5889a5ef3e97522ea5
Meeting-id: 121 091 0310
Password: Rgp9pA2wHW2
Gastgeber: Aswathy Velutharambath, Roman Klinger
Current evaluation of the generalization of natural language processing (NLP) systems, and much of machine learning, primarily consists of measuring the accuracy on held-out instances of the dataset. Since the held-out instances are often gathered using similar annotation process as the training data, they include the same biases that act as shortcuts for machine learning models, allowing them to achieve accurate results without requiring actual natural language understanding. Thus held-out accuracy is often a poor proxy for measuring generalization, and further, aggregate metrics have little to say about where the problem may lie.
In this talk, I will introduce a number of approaches we are investigating to perform a more thorough evaluation of NLP systems. I will first provide an overview of automated techniques for perturbing instances in the dataset that identify loopholes and shortcuts in NLP models, including semantic adversaries and universal triggers. I will then describe recent work in creating comprehensive and thorough tests and evaluation benchmarks for NLP that aim to directly evaluate comprehension and understanding capabilities. The talk will cover a number of NLP tasks, including sentiment analysis, textual entailment, paraphrase detection, and question answering.
About the speaker: Dr. Sameer Singh is an Assistant Professor of Computer Science at the University of California, Irvine (UCI). He is working primarily on robustness and interpretability of machine learning algorithms, along with models that reason with text and structure for natural language processing. Sameer was a postdoctoral researcher at the University of Washington and received his PhD from the University of Massachusetts, Amherst, during which he also worked at Microsoft Research, Google Research, and Yahoo! Labs. He was selected as a DARPA Riser, and has received the NSF CAREER award, UCI ICS Mid-Career Excellence in research award, and the Hellman and the Noyce Faculty Fellowships. His group has received funding from Allen Institute for AI, Amazon, NSF, DARPA, Adobe Research, Base 11, and FICO. Sameer has published extensively at machine learning and natural language processing venues, including paper awards at KDD 2016, ACL 2018, EMNLP 2019, AKBC 2020, and ACL 2020. You can find more information on his website: http://sameersingh.org/
Dallas Card (Stanford University):
Challenges and Opportunities for Evaluating Progress in NLP
Montag, 31. Mai 2021, 17.30 Uhr, über WebEx:
https://unistuttgart.webex.com/meet/gabriella.lapesa
Gastgeberin: Gabriella Lapesa
The past few years have seen remarkable advances in NLP, both in terms of performance on benchmark tasks and in the increasing prominence of real world systems. In assessing such progress, however, we need to ask not only what system achieves the best performance, but how it does so, how much we can trust the evaluation, and what the consequences of deploying such a system might be. In the first part of this talk, I will focus on the use of empirical evaluation in NLP research, presenting work on the influence of hyperparameter tuning and the importance of statistical power, along with some suggestions for best practices. In the second part, I will present a more philosophical discussion of the idea of fairness, and raise some broader difficulties around how to think about creating socially beneficial NLP systems.
Bio: Dallas Card is a postdoctoral researcher in the NLP group and the Data Science Institute at Stanford University. His work focuses on making machine learning more reliable and responsible, and on computational social science using textual data. Dallas is a graduate of Systems Design Engineering at the University of Waterloo and holds a PhD in machine learning from Carnegie Mellon University..
Thibault Clérice (Ecole nationale des Chartes, Paris):
Building an infrastructure for annotating medieval and classical languages
Mittwoch, 2. Juni 2021, 11.30 Uhr, über WebEx:
https://unistuttgart.webex.com/meet/mathilde.regnault
Gastgeberin: Mathilde Regnault
Annotating corpora with lexical information as well as morpho-syntactical ones is a tedious and costly task. Given the need expressed by researchers at the École nationale des Chartes and their colleagues in other institutions, we started building software bricks and corpora which became, over the years, a real ecosystem composed of corpora, taggers, web services and applications. In this paper, we seek to describe, explain and evaluate the design of the resulting environment as well as evaluating its costs, its missing bits, its failures (specifically what we should have done differently) and, of course, its favorable outcomes.
Natasha Korotkova (University of Konstanz):
Find, must, and conflicting evidence (joint work with Pranav Anand)
Dienstag, 8. Juni 2021, 17.30 Uhr, über WebEx:
https://unistuttgart.webex.com/meet/judith.tonhauser
Gastgeber: Daniel Hole, Judith Tonhauser
Find-verbs - English "find", German "finden", French "trouver" and their counterparts in other languages - have figured prominently in the literature on subjective language, as they only allow complements that are about matters of opinion, rather than fact. This talk focuses on a lesser-studied property of find-verbs: the ban on must-modals in their complements and their interaction with epistemics and evidentials at large. While it has been previously attributed to a clash in subjectivity, it will be argued instead that the find-must ban is of evidential nature: find-verbs convey directness, must-modals convey indirectness, and their combination is a semantic contradiction. Find-verbs will be shown to ban a variety of indirect markers across languages and to allow certain epistemic modals, but only those that do not semantically encode indirectness.
Loïc Grobol (LLF, Université de Paris):
La Queste del Analyseur: leaving no data untouched to parse Old French
Mittwoch, 16. Juni 2021, 11.30 Uhr, über WebEx:
https://unistuttgart.webex.com/meet/mathilde.regnault
Gastgeberin: Mathilde Regnault
Automatic syntactic parsing has seen tremendous improvements in the last years, driven by the conjunction of several factors: the advent of neural networks-based parsers, and in particular of *end-to-end* models ; the introduction of new representation techniques that do not necessitate access to annotated resources ; and the work of multilingual data production, aggregation and standardization in the Universal Dependencies project. Old French, by convention the earliest state of French for which written documents exist, has always been in an interesting position from a linguistic point of view, but these recent advances put it in an even more interesting position for natural language processing. The existence of a large annotated treebank, the Syntactic Reference Corpus of Medieval French (SRCMF), and its close relatedness to contemporary French and other well-resourced Romance languages make it suitable to the most recent automatic parsing techniques. On the other hand, its high variability (both due to the intrinsic variability of the language and to corpus sampling effects) and the inescapable scarcity of raw linguistic data makes it particularly challenging.
In this talk, I will present the PROFITEROLE (PRocessing Old French Instrumented TExts for the Representation Of Language Evolution) project, its goals and its means and discuss our recent works on statistical parsing of Old and Middle French. We experiment with different techniques to leverage as much of the available linguistic data — annotated or not — as possible that could be relevant for this task. We show that, while scarce, the Old French data that can currently be gathered are already sufficient to train valuable parsers but also that using contemporary French resources can yield even further improvements and provides surprisingly robust models.
Vivek Srikumar (University of Utah):
Adventures with Neural Networks and Logic: Semantic Roles and Beyond
Montag, 21. Juni 2021, 17 Uhr, über WebEx:
https://unistuttgart.webex.com/meet/erenay.dayanik
Gastgeber: Erenay Dayanik
Zusammenfassung: Today's dominant approach for modeling complex tasks involving human language calls for training neural networks using massive datasets. While the agenda is undeniably successful, we may not have the luxury of annotated data for every task or domain of interest. Reducing dependence on labeled examples may require us to rethink how we supervise models.
In this talk, I will use the task of semantic role labeling to motivate and explore this problem. We will look at recent work allows us to use knowledge to inform neural networks, without introducing additional parameters. Declarative rules stated in logic can be systematically compiled into computation graphs that augment the structure of neural models, and also into regularizers that can use labeled or unlabeled examples. I will present experiments which show that such declaratively constrained neural networks are not only more accurate, but also more consistent in their predictions.
Bio: Vivek Srikumar is associate professor in the School of Computing at the University of Utah. His research lies in the areas of natural language processing and machine learning and has been driven by questions arising from the need to reason about textual data with limited explicit supervision and to scale NLP to large problems. His work has been published in various AI, NLP and machine learning venues and has been recognized by paper awards/honorable mention from EMNLP and CoNLL. His work has been supported by awards from the National Science Foundation, and also from Google, Intel and Verisk. He obtained his Ph.D. from the University of Illinois at Urbana-Champaign in 2013 and was a post-doctoral scholar at Stanford University.
Evelyn Gius (Universität Darmstadt):
Literature as Data: A Literary Studies Perspective on Computational Text Analysis
Dienstag, 12. Juni 2021, 17.30 Uhr, über WebEx
Gastgeberin: Kerstin Jung
Veranstaltung im Rahmen der SDC4Lit Lecture Series
Gastgeberin: Mathilde Regnault
Gastgeber: Daniel Hole, Judith Tonhauser
Carolin Odebrecht (HU Berlin):
Challenges for Data Curation and Selection. Starting Infrastructure Community for Computational Literary Studies
Dienstag, 13. Juli 2021, 17.30 Uhr, über WebEx
Gastgeberin: Kerstin Jung
Veranstaltung im Rahmen der SDC4Lit Lecture Series
Wintersemester 2020
Andrey Kutuzov (Oslo):
Contextualised embeddings for semantic change detection: lessons learned
Montag, 7. Dezember 2020, 14 Uhr, über WebEx:
https://unistuttgart.webex.com/meet/dominik.schlechtweg
Gastgeber: Dominik Schlechtweg, Sabine Schulte im Walde
Contextualized embedding-based methods for diachronic semantic change detection are gaining traction now, but still lack thorough analysis of their predictions. We will talk about an attempt for such qualitative analysis of the semantic change scores predicted for English words across 5 decades. In particular, we will show that contextualized methods can sometimes predict high change scores for words which are not undergoing any real diachronic semantic shift. The reason for that is that these architectures confound changes in lexicographic senses and changes in contextual variance, which naturally stems from their distributional nature. Additionally, they often merge together syntactic and semantic aspects of lexical entities. Such cases will be discussed in detail with examples, their linguistic categorization and possible solutions.
Eva Wittenberg (UC San Diego):
Deverbal zero derivations from a psycholinguistic perspective
Dienstag, 15. Dezember 2020, 15.45 Uhr, über WebEx:
https://unistuttgart.webex.com/meet/daniel.hole
Gastgeber: Daniel Hole, Gianina Iordăchioaia, Judith Tonhauser
Deverbal zero derivations, such as "hug", "kick", or "nap", pose many a puzzle: How do children learn the meaning of nouns that look like verbs? How, if at all, do these nouns deploy the argument structure associated with the events they encode in a complex predicate? And how do we, as theory-building linguists, make sense of categorically flexible words in linguistic architecture? In this talk, I review experimental evidence from the language acquisition literature, and my own work on how adults process complex predicates and make sense of them, and I discuss what (I think) the data mean in light of how words connect to meaning in our minds.
Andres Karjus (Tallinn):
Exploring lexical dynamics using diachronic corpora and artificial language experiments
Montag, 11. Januar 2021, 14 Uhr, über WebEx:
https://unistuttgart.webex.com/meet/sabine.schulte-im-walde
Gastgeber: Dominik Schlechtweg, Sabine Schulte im Walde
Diachronic text corpora, both those spanning centuries and those mined from the web over shorter time spans, enable a usage-based approach to the study of evolutionary dynamics in languages, supported by advances in natural language processing for quantifying meaning (cf. Hamilton et al. 2016, Dubossarsky et al. 2019, Turney et al 2019). In this talk, I introduce recent work on inferring lexical competition dynamics and topical shifts from corpora of multiple languages and genres, but also a complimentary communication experiment designed to probe individual-level lexification processes. This all is driven by an overarching hypothesis is that language change is often enough driven by changes in the speakers’ communicative needs (in the sense of Winters et al. 2015, Regier et al. 2016, Kemp et al 2018). The artificial language communication experiment was designed to investigate a related claim concerning lexification dynamics in a recent cross-linguistic study (Xu et al. 2020). The experiment replicates the typological tendencies but also provides support for the communicative need hypothesis. I will argue that combining these different approaches – population-level information from diachronic corpora, typological data, and experiments with human participants – allows for a more complete picture of language change to be revealed than any one method alone could.
Edgar Onea (University of Graz):
On topic. Rethinking topic as part of argument structure
Dienstag, 26. Januar 2021, 15.45 Uhr, über WebEx:
https://unistuttgart.webex.com/meet/daniel.hole
Gastgeber: Daniel Hole, Judith Tonhauser
Usually, the notion of topicality is considered a foundational part of information structure, a layer of grammar that is clearly to be distinguished from argument structure. The reasoning for this insight is very straightforward: the very same verb with the very same arguments can – depending on e.g. word order or some additional morphology – exhibit different topics. (1) is a simple example.
(1) a. As for Melinda, Bill loves her.
(1) b. As for Bill, he loves Melinda.
In this talk I argue that such data have been fundamentally misinterpreted which hindered progress for many years. Here is my main point. When paraphrasing the two utterances in (1) in reported speech, we do in fact use a PP to mark the topic in the usual argument-structural way, as shown in (2).
(1) a. Alfred told me about Melinda that Bill loves her.
(2) b. Alfred told me about Bill that Melinda loves her.
Notice, first, that such effects cannot be reproduced consistently with focus (focus is transparent in embedding). More importantly, the data in (2) show that the about-PP appears NOT to be part of the argument structure of the embedded verb but of the matrix verb. Thus, I will suggest, what goes wrong in the classical analysis of (1) is that we think of the extraposed topic as orthogonal to the argument structure of the wrong verb: topics are in fact arguments of the covert performative speech act verb that takes the entire CP as an argument. Thus, what actually happens in (1a) is best approximated by (3).
(3) I hereby assert about Melinda, that bill loves her.
With this shift in perspective, I focus on three questions: a) Are there truth conditional effects associated with topic? b) Are there argument alternations with topic? and c) What is the semantic of topics?
I argue that topic is a semantic role of a limited set of events. These events also need to have a content. Topics provide an abstract pattern for the interpretation of that event which may result in truth conditional effects for attitude verbs like complain or surprise but not for plain speech act verbs such as say or tell. The truth conditional topic transparency of all verbs that can be 0-performatives in the structure of assertions is precisely the reason why the received wisdom that topics are in fact truth conditionally innocent could ever emerge. Moreover, I discuss a range of data showing how classical argument alternations with topics appear in various languages.
Laure Sarda (CNRS, Lattice):
Motion verbs: revisiting the manner/result complementarity in light of French data
Mittwoch, 27. Januar 2021, 11.30 Uhr, über WebEx:
https://unistuttgart.webex.com/meet/mathilde.regnault
Gastgeberin: Mathilde Regnault
Vlad Niculae (University of Amsterdam):
Learning with Sparse Latent Structure
Montag, 1. Februar 2021, 14 Uhr, über Webex.
Meeting-id: 121 366 9032
Password: NXssAZCN285
Gastgeber: Roman Klinger, Laura Oberländer
Structured representations are a powerful tool in machine learning, in particular for natural language: The discrete, compositional nature of words and sentences leads to natural combinatorial representations such as trees, sequences, segments, or alignments, among others. Such representations are at odds with deep neural networks, which conventionally perform smooth, soft computations, learning dense, inscrutable hidden representations.
We present SparseMAP, a strategy for inferring differentiable combinatorial latent structures, alleviating the tension between discrete and continuous representations through sparsity. SparseMAP computes a globally-optimal combination of a very small number of structures and can be extended to arbitrary factor graphs (LP-SparseMAP), only requiring access to local maximization oracles. Our strategy is fully deterministic and compatible with standard gradient-based methods for training neural networks. We demonstrate sparse and structured neural hidden layers, with successful empirical results and visualization properties.
Joint work with Gonçalo M Correia, Tsvetomila Mihaylova, Andre FT Martins, Mathieu Blondel, Claire Cardie, Wilker Aziz.
The speaker
Dr. Vlad Niculae [he/him] is an assistant professor in the Language Technology Lab, part of the Informatics Institute at the University of Amsterdam. Vlad’s research lies at the intersection of machine learning and natural language processing, building upon techniques from optimization, geometry, and probability to develop and analyze better models of language structures and phenomena. He obtained his PhD in Computer Science from Cornell University in 2018 and has worked until 2020 as a post-doctoral researcher in the DeepSPIN project (Deep Structured Prediction for Natural Language Processing) at the Instituto de Telecomunicações, Lisbon, Portugal.
Please find more information on his website: https://vene.ro/
Sommersemester 2020
Ryan Bochnak (University of Manchester):
Towards a Semantics of Graded Futures in Washo
Dienstag, 12. Mai 2020, 17:30 Uhr, über WebEx
https://unistuttgart.webex.com/meet/judith.tonhauser
Gastgeber: Daniel Hole, Judith Tonhauser
This talk discusses the semantics of the graded future morphemes -ašaʔ (“near future”), -tiʔ (“intermediate future”) and -gab (“distant future”) in Washo (isolate, USA; Jacobsen 1964). Several diagnostics set -ašaʔ (“near”) apart from the other two. First, -ašaʔ occurs in a different morphological slot in the verbal complex than the other two. Second, -ašaʔ does not directly encode temporal remoteness. In contexts where speakers have no idea when an event will take place in the future (a couple hours from now, or next week), speakers consistently use -ašaʔ, and not the more specific forms. Third, -tiʔ and -gab are constrained to appear only in specific licensing environments, but -ašaʔ is not. Specifically, -tiʔ and -gab can only occur in the scope of an overt modal or attitude verb, in questions, or in conditionals. Two challenges present themselves for the analysis of the Washo system. First, how does the “near future” inference for -ašaʔ arise? On the one hand, it seems natural to assume that -ašaʔ gives rise to a “near future” inference due to competition with -tiʔ and -gab, which are each more specific. On the other hand, many current theories of pragmatic competition rely on the notion of structural parallelism (e.g., Katzir 2007), whereas the Washo futures are not members of the same morphological paradigm. The second challenge is characterizing the licensing environments for -tiʔ and -gab. Although the notions of modality (Matthewson 2012) and veridicality (Mucha 2016) have been argued to play a role in licensing futures in other languages, neither notion fully covers the licensing environments for -tiʔ and -gab. Nevertheless, the fact that -tiʔ and -gab require licensing corroborates the view that future meaning is decomposable into temporal and modal/“extra” components (e.g., Kratzer 2012, Matthewson 2012).
Martina Wiltschko (Barcelona):
Beyond truth. The view from response markers.
Dienstag, 26. Mai 2020, 17:30 Uhr, über WebEx
https://unistuttgart.webex.com/meet/eleonore.brandner
Gastgeberin: Ellen Brandner
Ashwini Deo (Ohio State University):
The strongest alternative: Marathi =c and its Indo-Aryan counterparts
Dienstag, 9. Juni 2020, 17:30 Uhr, über WebEx
https://unistuttgart.webex.com/meet/judith.tonhauser
Gastgeber: Daniel Hole, Judith Tonhauser
Several Indo-Aryan languages contain a discourse clitic whose uses overlap with those of distinct English discourse particles like exclusive only, mirative just, emphatic right, intensifier absolutely, and scalar additive even without corresponding perfectly to either of them. This clitic (realized as =(a)c in Marathi, =i in Bangla, =j in Gujarati, and =hi in Hindi), exhibits a range of functions that are not clustered together in any known discourse marker in Germanic or Romance. Given that our understanding of the semantic typology of discourse particles is heavily based on those that are instantiated in European languages, Marathi =c and its semantic cognates in Indo-Aryan pose a puzzle regarding possible lexicalizations of these meanings cross-linguistically.
The challenge for a unified semantic analysis is to derive the availability of this full range of uses from a single lexical entry in interaction with linguistic and extra-linguistic contextual information. I propose an analysis that does this by extending the analytical tools from Beaver & Clark (2008), Coppock & Beaver (2011), and Velleman et al (2013). These analyses assume that the answers to the Current Question (CQ) are ranked by 'strength', where strength may be understood as entailment-based (stronger alternatives entail weaker alternatives) or given by a contextually accessed scale. Focus-sensitive discourse particles are taken to comment on the strength relation between the prejacent p and alternatives p’ in the CQ at a given context. An exclusive like only, for instance, is analyzed as conveying that the prejacent is the strongest among all alternatives that are true at the world of evaluation. I suggest that it is also possible to compare the strength of alternative propositions that vary only with respect to how strictly context-sensitive expressions in a given sentence are interpreted. Such a set of alternatives will also form an entailment scale. Once this sort of interpretation-based ranking is introduced, the varied uses of Marathi =c and its Indo-Aryan semantic cognates) fall out naturally from the nature of the scale along which alternatives in the CQ are ranked. In providing an analysis for Marathi =c by conservatively extending already existing tools, I attempt to further our understanding of the semantic ingredients that are relevant to describing the cross- linguistic inventory of discourse expressions.
Andres Karjus (University of Edinburgh)
Montag, 29. Juni 2020, 14:00 Uhr verschoben
Gastgeber: Dominik Schlechtweg, Sabine Schulte im Walde
Britta Stolterfoht (Tübingen):
Processing Temporality: Position, Tense and Aspect
Dienstag, 14. Juli 2020, 17:30 Uhr, über WebEx
https://unistuttgart.webex.com/meet/judith.tonhauser
Gastgeberin: Judith Tonhauser
Frühere Semester
Datum | Zeit | Vortragender & Titel | Raum | Gastgeber |
12.11.2019 | 15.45 | Ryan Bochnak (University of Manchester): Towards a Semantics of Graded Futures in Washo |
cancelled | Daniel Hole, Judith Tonhauser |
19.11.2019 | 15.45 | Gereon Müller (Universität Leipzig): Syntactic Strength: A New Approach |
K2, M 17.25 | Daniel Hole, Judith Tonhauser |
26.11.2019 | 15.45 | Jaklin Kornflint (Syracuse University): NP versus DP: How successful a parameter is it cross-linguistically? |
K2, M 17.25 | Daniel Hole, Judith Tonhauser |
10.12.2019 | 15.45 | Beste Kamali (Universität Bielefeld): Polar Questions meet Focus Projection: A view from Turkish |
K2, M 17.25 | Daniel Hole, Judith Tonhauser |
14.01.2020 | 15.45 | Anne Mucha (IDS Mannheim): The temporal interpretation of complement clauses: Cross-linguistic and experimental data |
K2, M 17.25 | Daniel Hole, Judith Tonhauser |
20.01.2020 | 11.30 | Björn Schuller (Universität Augsburg): Computational Paralinguistics: Season 3, Episode 1 |
FZI, V 5.01 | Michael Neumann |
27.01.2020 | 14.00 | Carolin Odebrecht (Humboldt-Universität Berlin) Handling the diversity of speech, texts and concepts created in experimental, data based or data driven research: Research data management in the context of a diversity in data and methods for literary studies and linguistics |
FZI, V 5.01 | Kerstin Jung |
29.01.2020 | 11.30 | Daniela Marzo (LMU München): Partizipien Perfekt und V>N-Konversion im (Alt-)Italienischen |
K2, M 17.25 | Gianina Iordăchioaia |
10.02.2020 | 14.00 | Rico Sennrich (Universität Zürich): Document-level Machine Translation: Recent Progress and The Crux of Evaluation |
FZI, V 5.01 | Michael Roth |
20.02.2020 | 14.00 | Martin Hilpert (Université de Neuchâtel): On the history of permissive get in American English: New quantitative evidence |
FZI, V 5.01 | Sabine Schulte im Walde |
Ryan Bochnak:
Towards a Semantics of Graded Futures in Washo
(Dienstag, 12. November 2019)
This talk discusses the semantics of the graded future morphemes -ašaʔ (“near future”), -tiʔ (“intermediate future”) and -gab (“distant future”) in Washo (isolate, USA; Jacobsen 1964). Several diagnostics set -ašaʔ (“near”) apart from the other two. First, -ašaʔ occurs in a different morphological slot in the verbal complex than the other two. Second, -ašaʔ does not directly encode temporal remoteness. In contexts where speakers have no idea when an event will take place in the future (a couple hours from now, or next week), speakers consistently use -ašaʔ, and not the more specific forms. Third, -tiʔ and -gab are constrained to appear only in specific licensing environments, but -ašaʔ is not. Specifically, -tiʔ and -gab can only occur in the scope of an overt modal or attitude verb, in questions, or in conditionals. Two challenges present themselves for the analysis of the Washo system. First, how does the “near future” inference for -ašaʔ arise? On the one hand, it seems natural to assume that -ašaʔ gives rise to a “near future” inference due to competition with -tiʔ and -gab, which are each more specific. On the other hand, many current theories of pragmatic competition rely on the notion of structural parallelism (e.g., Katzir 2007), whereas the Washo futures are not members of the same morphological paradigm. The second challenge is characterizing the licensing environments for -tiʔ and -gab. Although the notions of modality (Matthewson 2012) and veridicality (Mucha 2016) have been argued to play a role in licensing futures in other languages, neither notion fully covers the licensing environments for -tiʔ and -gab. Nevertheless, the fact that -tiʔ and -gab require licensing corroborates the view that future meaning is decomposable into temporal and modal/“extra” components (e.g., Kratzer 2012, Matthewson 2012).
Gereon Müller:
Syntactic Strength: A New Approach
(Dienstag, 19. November 2019)
If relative strength of syntactic categories is viewed as a core concept of grammar (e.g., to account for phenomena like pro-drop, complementizer-trace effects, and V-to-T movement; see Chomsky (2017) for a recent appraisal), an approach to syntax is ultimately required where the elementary building blocks of grammar (rules, operations, constraints, etc.) are sensitive to the strength of categories. Gradient Harmonic Grammar (Smolensky & Goldrick (2016)) is a new approach where different weights are associated both with grammatical constraints (as in standard Harmonic Grammar; Pater (2016)) and, crucially, with syntactic categories; closer inspection reveals that a direct predecessor of this theory is Squishy Grammar as developed by Ross (1973; 1975). In this talk, I argue that a strength-based approach to syntactic categories can shed new light on some well-known problems raised by asymmetries in movement operations in German, among them (i) asymmetries between movement types with respect to local domains (e.g., scrambling cannot leave a finite CP, wh-movement can do so); (ii) asymmetries between moved items with respect to local domains and movement types (e.g., subjects and objects can both undergo long-distance wh-movement from a declarative CP, but only objects can undergo long-distance topicalization from a wh-island; regular objects can undergo topicalization, scrambling and wh-movement from VP but objects that are part of semantically fully opaque idioms can only undergo topicalization (`Vorfeldbesetzung') from VP, not scrambling or wh-movement); and (iii) asymmetries between local domains (e.g., VP is a barrier for fewer movement operations across it than CP, and a non-finite restructuring CP is a barrier for fewer movement operations than, say, a finite CP).
More generally, if such an approach proves tenable, it provides a simple approach to a classical conundrum: On the one hand, there is evidence for fine-grained systems of various functional heads in each of the CP, TP, and vP domains; on the other hand, for most syntactic purposes, the additional heads behave as if they were not present. In the present approach, the dilemma can be solved by attributing very little strength to most of these items in any given language.
Beste Kamali:
Polar Questions meet Focus Projection: A view from Turkish
(Dienstag, 26. November 2019)
The Turkish polar question clitic -mI attaches to a focused constituent and forms Polar Questions comparable in meaning to a cleft question in English depending on its attachment site. For instance, attached to the subject, it yields a question like 'Was it JOHN who made dinner?'. I will discuss in this talk the systematic contrasts between PQs with a verbal clitic and those with what I will show to be a VP-second clitic, both of which are licensed in all-new contexts and reflect ``broad focus". I will argue that verbal attachment expresses Hamblin-style alternatives while VP-second attachment yields diverse, non-negated propositional alternatives via focus projection, which I model in Commitment Space Semantics (Krifka 2014).
Anna Mucha:
The temporal interpretation of complement clauses: Cross-linguistic and experimental data
(Dienstag, 14. Januar 2020)
The talk will start out from the well-known observation that in English, past-marked stative clauses embedded under past-marked attitude verbs as in (1) can receive two temporal interpretations: backward-shifted (1-a) or simultaneous (1-b).
(1) Mary said [that John was sick].
- a. Mary said: `John was sick.' (backward-shifted reading)
- b. Mary said: `John is sick.' (simultaneous reading, "Sequence of Tense")
Under an analysis in which the difference between (1-a) and (1-b) amounts to structural ambiguity (e.g., Ogihara 1995, 1996; Stowell 1995; Kusumoto 1999, 2005), the complement clause in (1) can be semantically tenseless, despite morphological tense marking. Languages in which the equivalent of (1) only has a backward-shifted reading (e.g., Japanese, Polish) would lack the structural licensing mechanism that allows the embedded past not to be interpreted.
The aim of the talk is twofold: The first part provides a broader cross-linguistic perspective on the temporal interpretation of complement clauses by discussing data from languages that do not obligatorily mark tense, and thus allow for morphologically tenseless complement clauses: Medumba, Washo, Hausa and Samoan (based on Bochnak, Hohaus and Mucha 2019). Cross-linguistic comparison reveals variation parallel to the difference between English and Japanese in languages with optional tense marking: In Medumba (Grassfields Bantu), past-marking entails semantic backward-shifting while in Washo (language isolate) it does not. Moreover, differences in the availability of backward-shifted and simultaneous interpretations of morphologically tenseless complement clauses between the four languages will be discussed. The second part of the presentation concerns the question of how sequence-of-tense variation in tensed languages should be analyzed. I will briefly introduce a pragmatic approach to SOT (Altshuler and Schwarzschild 2013; Altshuler 2016) as a potential alternative to structural analyses that assume semantic tenselessness to be the source of simultaneous interpretations. Then I will present ongoing work on Polish (in collaboration with Agata Renans and Jacopo Romoli) that explores the empirical predictions of these different approaches to (non-)SOT variation.
Björn Schuller:
Computational Paralinguistics: Season 3, Episode 1
(Montag, 20. Januar 2020)
Computational Paralinguistics have come a long way from the identification of the speaker and her gender via emotion and stress to our current days of rich computational speaker state and trait assessment. Roughly, one could divide the time since its rise into two periods: First are the earlier days, dominated by mostly lab recorded data, traditional expert-designed features, and simple machine learning algorithms. The following era - starting roughly in the later naughties - was marked by more and more moving into "the wild“ in terms of data-realism, self-learning of feature representations, and increasingly deep and more complex machine learning solutions. In both eras, however, the focus was largely on improving recognition performances as prime or sole target, while broadening the beam of speaker states and traits considered, and gently moving into more realistic data settings, albeit still operating at small scale, and learning largely if not entirely supervised. From this point of view, one may argue that we are starting to move into a third age, which targets "holistic" paralinguistics at scale in real-world applications. This age’s four horsemen include 1) an increasing interest in „green“, i.e., efficient, 2) explainable paralinguistics realised 3) in closed-loop generation and analysis, and 4) gradually learnt also reinforced. From an apocalyptic "big brother"-esque view point of which dangers come with computers soon being able to "know it all“ about us from our voice and language arise new challenges: rendering computational paralinguistics safe against attacks, and offering to protect speakers from an increasingly super-human performance able to soon recognise even suppressed or pretended states and traits will become a core responsibility of the research community that breathed life into the field at first. In this talk’s director’s cut, aiming at inducing considracy, we shall reflect how to best meet the "A.I. can be dangerous“ cliff-hanger that marked the last episode of Season 2 in Computational Paralinguistics - be ready for Season 3’s opening.
Carolin Odebrecht:
Handling the diversity of speech, texts and concepts created in experimental, data based or data driven research: Research data management in the context of a diversity in data and methods for literary studies and linguistics
(Montag, 27. January 2020)
Many (not only) humanities departments have already been made sensitive to the tasks and challenges of the research data management by the funding agencies, university initiatives and their own research projects. However, it is a truism that the awareness of such “meta topics” is sufficient to actually address the disciplines needs concerning data creation and handling, storage, publication or documentation. Especially, the literary studies and linguistics are characterized by an enormous amount of data and methodological diversity. With this circumstance, there can hardly be a general approach to research data management. Thus, research data management should always be accompanied by a domain-, data- or approach-specific perspective.
How can we ensure a balance between general management requirements and scientific research interest that allows empirical, data based, small or large-scale research projects and does not prevent this due to a lack of external expertise and capacities? In my talk, I present the approach of the Faculty of Language, Literature and Humanities of HU Berlin, which focuses on a domain-specific approach on research data management. In addition, I would like to discuss this interdisciplinary and administratively complex meta-topic.
Daniela Marzo:
Partizipien Perfekt und V>N-Konversion im (Alt-)Italienischen
(Mittwoch, 29. Januar 2020)
Der Vortrag hat es zum Ziel, die Rolle der Partizipien Perfekt für die V>N-Konversion im (Alt-)Italienischen neu zu definieren. Es wird dabei die Hypothese diskutiert, dass weit mehr der deverbalen italienischen Nomen als bislang gedacht auf der Grundlage von Partizipien Perfekt gebildet sind. Ausgangspunkt der Überlegungen ist die Beobachtung, dass viele Verben des Altitalienischen über im modernen Italienischen nicht mehr gebräuchliche kurze (auch starke oder unregelmäßige) Partizipien Perfekt verfügen, die homonym zu entsprechenden Nomen sind (z.B. guastoPart ʻbeschädigtʼ vs. guastoN ʻSchadenʼ).
Auf der Grundlage von Korpusdaten sollen Anhaltspunkte für die Existenz eines im Altitalienischen produktiven Wortbildungsmusters gegeben werden, das im modernen Italienischen nur noch in Relikten aktiv ist. Dabei soll anhand semantischer und syntaktischer Kriterien erörtert werden, ob es sich um Konversion der Wurzel, eines spezifischen Verbstammes oder aber um die Konversion der kompletten Partizipialform handelt.
Rico Sennrich:
Document-level Machine Translation: Recent Progress and The Crux of Evaluation
(Montag, 10. Februar 2020)
Machine translation (MT) is still predominantly modelled and evaluated on the level of sentences, but neural methods have the potential to overcome this limitation and allow effective document-level modelling. However, practical challenges of document-level MT include the lack of suitable training data, the high computational cost of wider-context models, and low reward for "context-aware" translation in automatic metrics. In my talk, I will discuss recent neural architectures that take into account wider context and address computational and data bottlenecks in different ways, and their evaluation with test sets that are targeted towards discourse phenomena. While evaluation with automatic metrics such as BLEU is noisy and hard to interpret, I will show that targeted evaluation can guide the development of document-level system by highlighting the effects of various modelling decisions.
Martin Hilpert (joint work with Florent Perek, University of Birmingham):
On the history of permissive get in American English: New quantitative evidence
(Donnerstag, 20. Februar 2020)
This talk investigates the diachronic development of permissive uses of the English verb get, as illustrated below:
(1) In the movies the prisoners always get to make one phone call.
Different views exist on how such uses emerged. Gronemeyer (1999: 30) suggests that the permissive meaning derives from causative uses (I got him to confess); van der Auwera et al. (2009: 283) view permissive get as an extension of its acquisitive meaning (I got a present). In this talk, we argue that permissive get evolved out of inchoative uses of get in a similar construction (e.g., You're getting to be a big girl now) that invited the idea of a permission, which eventually conventionalized.
Drawing on data from the COHA (Davies 2010) between 1860 and 2009, we find a substantial diachronic increase of permissive get, which is driven by verbs such as see, be, meet:
(2) I guess we won't get to see Colonel Morrison after all. (1910s)
(3) Some day she'd get to be an editor herself. (1930s)
(4) Oh thank you and you'll get to meet our new minister then sure! (1900s)
Early examples are non-agentive and compatible with the inference of a permission. Later uses include a wider set of lexical verbs with agentive meanings, which thus encode actions that can be permitted.
We report further evidence for the diachronic relation between the permissive and inchoative uses from a characterization of the semantic domain covered by the two constructions by means of a distributional semantic model, which captures semantic similarity between verbs through their co-occurrence frequency with other words (Lenci 2008). The pairwise distributional distance scores calculated from the model are used to place verbs in a visual representation. By creating different plots for the permissive and inchoative uses at several points in time, it is possible to compare the distribution of the two constructions from a semantic perspective, and to highlight whether and when they overlap.
We find that in the late 19th century the semantic domain of the inchoative use still largely overlaps with that of the permissive use, although the latter is already wider. Over time, the overlap becomes relatively smaller, as the permissive use sees a sharp increase in type frequency and expands into increasingly diverse semantic areas. We argue that these results illustrate the common origin of these constructions as well as the later emancipation of the permissive use, marked in particular by an increase of productivity, as is typically found for newly grammaticalised constructions.
References:
Davies, Mark. 2010. The Corpus of Historical American English (COHA): 400+ million words, 1810-2009. http://corpus.byu.edu/coha.
Gronemeyer, Claire. 1999. On deriving complex polysemy: The grammaticalization of get. English Language and Linguistics 3. 1–39.
Lenci, Alessandro. 2008. Distributional semantics in linguistic and cognitive research. Rivista di Linguistica 20(1). 1–31.
van der Auwera, Johan, Petar Kehayov and Alice Vittrant 2009. Acquisitive modals. In Hogeweg, L., de Hoop, H. and Malchukov, A. (eds.), Cross-linguistic Semantics of Tense, Aspect and Modality. Amsterdam. John Benjamins, 271–302.
Datum | Zeit | Vortragender & Titel | Raum | Gastgeber |
16.04.2019 | 11.30 | Massimo Poesio (Queen Mary University, London): Disagreements in anaphoric interpretation |
PWR 07, V7.22 | Diego Frassinelli |
29.04.2019 | 14.00 | R. Harald Baayen (Universität Tübingen): Throwing off the shackles of the morpheme with simple linear transformations |
FZI, V5.02 | Diego Frassinelli |
06.05.2019 | 14.00 | Marco del Tredici (University of Amsterdam): You Shall Know a User by the Company It Keeps: Dynamic Representations for Social Media Users in NLP |
FZI, V5.02 | Dominik Schlechtweg, Sabine Schulte im Walde |
13.05.2019 | 14.00 | Talitha Anthonio (University of Groningen): Different document representations in hyperpartisan news detection |
FZI, V5.02 | Michael Roth |
14.05.2019 | 15.45 | Andrew Koontz Garboden (University of Manchester): State/change of state polysemy and the lexical semantics of property concept lexemes |
K2, 17.21 | Gianina Iordachioaia |
20.05.2019 | 14.00 | Caroline Féry (Universität Frankfurt): Verum focus and sentence accent |
FZI, V5.02 | Sabrina Stehwien |
24.06.2019 | 14.00 | Rochelle Lieber (University of Hampshire): Modeling nominalization in the Lexical Semantic Framework |
K2, 17.92 | Gianina Iordachioaia |
25.06.2019 | 11.30 | Nina Tahmabesi (University of Gothenburg): On Lexical Semantic Change and Evaluation |
FZI, V5.02 | Dominik Schlechtweg, Sabine Schulte im Walde |
10.07.2019 | 14.00 | Ivan Vulic (University of Cambridge): Are Fully Unsupervised Cross-Lingual Word Embeddings Really Necessary? |
FZI, V5.01 | Diego Frassinelli |
Massimo Poesio (in Zusammenarbeit mit Jon Chamberlain, Silviu Paun, Alexandra Uma, Juntao Yu, Derya Cokal, Janosch Haber, Richard Bartle and Udo Kruschwitz):
Disagreements in anaphoric interpretation
(Dienstag, 16. April 2019)
The assumption that natural language expressions have a single, discrete and clearly identifiable meaning in a given context, successfully challenged in lexical semantics by the rise of distributional models, nevertheless still underlies much work in computational linguistics, including work based on distributed representations. In this talk I will first of all present the evidence that convinced us that the assumption that a single interpretation can always be assigned to anaphoric expression is no more than a convenient idealization. I will then discuss recent work on the DALI project that aims to develop a new model of interpretation that abandons this assumption for the case of anaphoric interpretaton / coreference. I will present the recently released Phrase Detectives 2.1 corpus, containing around 2 million crowdsourced judgments for more than 100,000 markables, an average of 20 judgments per markable; the Mention Pair Annotation (MPA) Bayesian inference model developed to aggregate these judgments; and the results of a preliminary analysis of disagreements in the corpus suggesting that between 10% and 30% of markables in the corpus appear to be genuinely ambiguous.
R. Harald Baayen:
Throwing off the shackles of the morpheme with simple linear transformations
(Montag, 29. April 2019)
Word and Paradigm Morphology (Blevins, 2016) has laid bare a series of foundational problems surrounding the post-Bloomfieldian theoretical construct of the morpheme as the minimal unit combining form and meaning. In my presentation, I will first provide an overview of these problems. I will then present a morpheme-free computational model of the mental lexicon, Linear Discriminative Learning (LDL), which implements central concepts of Word and Paradigm Morphology. LDL makes use of simple linear transformations between vectors in a form space and vectors in a semantic space to model lexical processing in comprehension and production. In the final part of this presentation, I will review a series of experimental findings that are traditionally interpreted as providing key evidence for morphemes, and I will show how these findings can be accounted for within the LDL framework.
References:
- Baayen, R. H., Chuang, Y. Y., Shafaei-Bajestan E., and Blevins, J. P. (2019). The discriminative lexicon: A unified computational model for the lexicon and lexical processing in comprehension and production grounded not in (de)composition but in linear discriminative learning. Complexity, 2019, 1-39.
- Baayen, R. H., Chuang, Y. Y., and Blevins, J. P. (2018). Inflectional morphology with linear mappings. The Mental Lexicon, 13 (2), 232-270.
- Blevins, J. P. (2016). Word and Paradigm Morphology. Oxford University Press
Marco del Tredici:
You Shall Know a User by the Company It Keeps: Dynamic Representations for Social Media Users in NLP
(Montag, 6. Mai 2019)
Information about individuals can help to better understand what they say, particularly in social media where texts are short. Current approaches to modelling social media users pay attention to their social connections, but exploit this information in a static way, treating all connections uniformly. This ignores the fact, well known in sociolinguistics, that an individual may be part of several communities which are not equally relevant in all communicative situations. In this talk I will present a new model based on Graph Attention Networks that captures this observation. The model dynamically explores the social graph of a user, computes a user representation given the most relevant connections for a target task, and combines it with linguistic information to make a prediction. I will present the results of our model on several downstream tasks in NLP, showing which are the advantages of dynamic representations for social media users over static ones.
Andrew Koontz Garboden (draws on collaborative work with: John Beavers (UT Austin), Ryan Bochnak (U Manchester), Margit Bowler (U Manchester), Mike Everdell (UT Austin), Itamar Francez (U Chicago), Emily Hanink (U Manchester), Kyle Jerro (U Essex), Elise LeBovidge (U Washington), and Stephen Nichols (U Manchester)):
State/change of state polysemy and the lexical semantics of property concept lexemes
(Dienstag, 14. Mai 2019)
As documented in the philosophical and linguistic literature (see e.g., Kennedy 2012 for an overview), there are classes of properties that hold of an individual not in an absolute fashion, but to some degree:
a. Kim is wiser than Sandy.
b. Sandy is taller than Kim.
c. Jo is happier than Jack.
The canonical lexicalization of such properties in English and many familiar languages is with adjectives. There are many lesser studied languages, however, in which the descriptive content expressed by English adjectives is more often lexicalized by nouns or verbs, as discussed extensively in the typological literature (Dixon 1982; Thompson 1989; Hengeveld 1992; Bhat 1994; Wetzer 1996; Stassen 1997; Beck 2002; Baker 2003). We follow Thompson (1989) in calling lexemes expressing this descriptive content `property concept lexemes' in recognition of the fact that crosslinguistically they lack a fixed category.
As part of a larger project investigating whether this variation in the category of property concept lexemes has any lexical semantic consequences, I report on preliminary research into the derivational relationship of property concept lexemes and change of state predicates. The English adjective `red' describes the state of being red while the verb `redden', derived from the adjective with the --en suffix, describes a change into that state. I show that in cases where the property concept lexeme is a verb, the verb is some times polysemous between a state and a change of state sense. Descriptions of changes into states lexicalized by adjectives or nouns, by contrast, show derivational morphology (e.g., English --en and allomorphs). I suggest that the possibility of verbal property concept lexemes to show a polysemy, by contrast with adjectival and nominal ones, is a consequence of only verbs being able to relate individuals to dynamic events, while adjectives and nouns cannot. I consider some of the virtues and complications of such a theory of the interface between lexical semantics and lexical category.
Caroline Féry:
Verum focus and sentence accent
(Montag, 20. Mai 2019)
If verum focus is to be analyzed as a Roothian kind of focus, eliciting a set of alternatives and following the regular rules of sentence accent assignment (Höhle 1992, Goodhue 2018), a dialogue like the following one is problematic since the only new element in Sam’s sentence is the negation.
Micah: Where is everyone else?
Sam: There IS noone else
However, the negation is not accented, instead the verb is accented (see Richter 1993 for a syntactic account of the unstressed status of the negation). In my talk, I will show that verum focus has a variety of additional interpretations (see Romero & Han 2004, Gutzmann & Castroviejo 2011, Lohnstein 2016, Samko 2017 among others) and I will introduce an additional one: counter-assertive vs. counter-presuppositional (Gussenhoven 1983). This phenomenon has been ignored so far in the literature on verum as far as I can tell: The counter-presuppositional interpretation of verum focus cancels a presupposition or a QUD at the same time as it introduces a verum focus.
Rochelle Lieber:
Modeling nominalization in the Lexical Semantic Framework
(Montag, 24. Juni 2019)
In this talk I start from the observation made in Lieber (2016) that English nominalizing affixes are almost always multiply polysemous. The specific case that I’ll focus on here is that of -ing nominalization, which, as Andreou and Lieber (in press) have shown, can express both eventive and referential readings, both count and mass quantification, and both bounded and unbounded aspect, with syntactic context playing a key role in determining the possible readings of any given nominalization. The apparent fact that nominalizers in English do not behave like “rigid designators”, as Borer (2013) has claimed, raises the question of how the construction of their readings can be modeled. I first provide a brief introduction to the Lexical Semantic Framework of Lieber (2004, 2016). I then show how LSF allows us to start with a lexical semantic representation of a nominalization that is radically underspecified, and how that underspecification can be resolved in context to give rise to readings that differ in eventivity, quantification, and aspectual reading.
Nina Tahmabesi:
On Lexical Semantic Change and Evaluation
(Dienstag, 25. Juni 2019)
In this talk I will give an overview of the work done in computational detection of semantic change over the past decade. I will present both lexical replacements and semantic change, and the impact these have on research in e.g., digital humanities. I will talk about the challenges of detecting as well as evaluating lexical semantic change, and our new project connecting computational work with high-quality studies in historical linguistics.
Ivan Vulic:
Are Fully Unsupervised Cross-Lingual Word Embeddings Really Necessary?
(Mittwoch, 10. Juli 2019)
Cross-lingual word representations offer an elegant and language-pair independent way to represent content across different languages. They enable us to reason over word meaning in multilingual contexts and serve as an integral source of knowledge for enabling language technology in low-resource languages through cross-lingual transfer. A current research focus is on resource-lean projection-based embedding models which require cheap word-level bilingual supervision. In the extreme, fully unsupervised methods do not require any supervision as all: this property makes such approaches conceptually attractive and potentially applicable to a wide spectrum of language pairs and cross-lingual scenarios. However, their only core difference to weakly supervised projection-based methods is in the way they obtain a seed dictionary used to initialize an iterative self-learning procedure. While the primary use case of fully unsupervised approaches should be low-resource target languages and distant language pairs, in this talk we show that even the most robust and effective fully unsupervised approaches still struggle in these challenging settings, often suffering from instability issues and yielding suboptimal solutions. What is more, we empirically demonstrate that even when fully unsupervised methods succeed, they never surpass the performance of weakly supervised methods (seeded only with 500-1,000 translation pairs) using the same self-learning procedure. These findings call for revisiting the main motivations behind fully unsupervised cross-lingual word embedding methods.
Datum | Zeit | Vortragender & Titel | Raum | Gastgeber |
13.09.2018 | 11.30 | Peter Turney (Independent Researcher): Natural Selection of Words: Finding the Features of Fitness |
FZI, V5.01 | Dominik Schlechtweg, Sabine Schulte im Walde |
07.11.2018 | 11.30 | Dan Jurafsky (Stanford University): Computational Extraction of Social Meaning from Language |
Informatik, V38.04 (Erdgeschoss) | Gabriella Lapesa |
09.11.2018 | 14.00 | Yadollah Yaghoobzadeh (Microsoft Research): Distributed Representations for Fine-Grained Entity Typing |
FZI, 02.026 | Thang Vu |
12.11.2018 | 14.00 | Diana McCarthy (University of Cambridge): Word Sense Models: From static and discrete to dynamic and continuous |
FZI, V5.02 | Dominik Schlechtweg |
13.11.2018 | 17.30 | Markus Steinbach (Universität Göttingen): Iconicity in narration. The linguistic meaning of gestures. |
K2, 17.24 | Daniel Hole |
26.11.2018 | 14.00 | Sven Büchel (Universität Jena): From Sentiment to Emotion: Challenges of a More Fine-Grained Analysis of Affective Language |
FZI, V5.02 | Roman Klinger |
29.11.2018 | 15.45 | Anders Søgard (University of Copenhagen): Hegel's Holiday: an Argument for a Less Empiricist NLP? |
FZI, V5.01 | Jonas Kuhn |
18.12.2018 | 17.30 | Judith Degen (Stanford University): On the natural distribution of "some" and "or": consequences for theories of scalar implicature |
K2, 17.24 | Judith Tonhauser |
28.01.2019 | 14.00 | Anna Hätty (Universität Stuttgart/BOSCH): The role of ambiguity, centrality and specificity for defining and extracting terminology (PhD Progress Talk) |
FZI, V5.02 | Sabine Schulte im Walde |
Peter Turney (in Zusammenarbeit mit Saif M. Mohammad):
Natural Selection of Words: Finding the Features of Fitness
(Donnerstag, 13. September 2018)
According to WordNet, clarity, clearness, limpidity, lucidity, lucidness, and pellucidity are synonymous; all of them mean free from obscurity and easy to understand. Google Books Ngram Viewer shows that clearness was, by far, the most popular member of this synset (synonym set) from 1800 to 1900 AD. After 1900, the popularity of clarity rose, surpassing clearness in 1934. By 1980, clarity was, by far, the most popular member of the synset and clearness had dropped down to the low level of lucidity. We view this competition among words as analogous to biological evolution by natural selection. The leading word in a synset is like the leading species in a genus. The number of tokens of a word in a corpus corresponds to the number of individuals of a species in an environment. In both cases, natural selection determines which word or species will dominate a synset or genus. Species in a genus compete for resources in similar environments, just as words in a synset compete to represent similar meanings. We present an algorithm that is able to predict when the leading member of a synset will change, using features based on a word’s length, its characters, and its corpus statistics. The algorithm also gives some insight into what causes a synset’s leader to change. We evaluate the algorithm with 9,000 synsets, containing 22,000 words. In a 50 year period, about 12 to 14 percent of the synsets experience a change in leadership. We can predict changes 50 years ahead with an F-score of 46 percent, whereas random guessing yields 14 to 19 percent. This line of research contributes to the sciences of evolutionary theory and computational linguistics, but it may also lead to practical applications in natural language generation and understanding. Evolutionary trends in language are the result of many individuals, making many decisions about which word to use to express a given idea in a given situation. A model of the natural selection of words can help us to understand how such decisions are made, which will enable computers to make better decisions about language use. Modeling trends in words will also be useful in advertising and in analysis of social networks.
Bio: Dr. Peter Turney is an independent researcher and writer in Gatineau, Quebec. He was a Principal Research Officer at the National Research Council of Canada (NRC), where he worked from 1989 to 2014. He was then a Senior Research Scientist at the Allen Institute for Artificial Intelligence (AI2), where he worked from 2015 to 2017. He has conducted research in AI for over 27 years and has more than 100 publications with more than 18,000 citations. He received a Ph.D. in philosophy from the University of Toronto in 1988, specializing in philosophy of science. He has been an Editor of Canadian Artificial Intelligence magazine, an Editorial Board Member, Associate Editor, and Advisory Board Member of the Journal of Artificial Intelligence Research, and an Editorial Board Member of the journal Computational Linguistics. He was the Editor of the ACL Wiki from 2006, when it began, up to 2017. He was an Adjunct Professor at the University of Ottawa, School of Electrical Engineering and Computer Science, from 2004 to 2015.
Dan Jurafsky:
Computational Extraction of Social Meaning from Language
(Mittwoch, 7. November 2018)
I give an overview of research from our lab on computationally extracting social meaning from language, meaning that takes into account social relationships between people. I'll describe our study of interactions between police and community members in traffic stops recorded in body-worn camera footage, using language to measure interaction quality, study the role of race, and draw suggestions for going forward in this fraught area. I'll describe computational methods for studying how meaning changes over time and new work on using these models to study historical societal biases and cultural preconceptions. And I'll discuss our work on framing, including agenda-setting in government-controlled media and framing of gender on social media. Together, these studies highlight how computational methods can help us interpret some of the latent social content behind the words we use.
Yadollah Yaghoobzadeh:
Distributed Representations for Fine-Grained Entity Typing
(Freitag, 9. November 2018)
Extracting information about entities remains an important research area. In this talk, I address the problem of fine-grained entity typing, i.e., inferring from a large text corpus that an entity is a member of a class, such as" food" or" artist". The application we are interested in is knowledge base completion, specifically, to learn which classes an entity is a member of. Neural networks (NNs) have shown promising results in different machine learning problems. Distributed representation (embedding) is an effective way of representing data for NNs. In this work, we introduce two models for fine-grained entity typing using NNs with distributed representations of language units: (i) A global model that predicts types of an entity based on its global representation learned from the entity’s name and contexts. (ii) A context model that predicts types of an entity based on its context-level predictions. Each of the two proposed models has specific properties. For the global model, learning high-quality entity representations is crucial. Therefore, we introduce representations on the three levels of entity, word, and character. We show that each level provides complementary information and a multi-level representation performs best. For the context model, we need to use distant supervision since there are no context-level labels available for entities. Distantly supervised labels are noisy and this harms the performance of models. Therefore, we introduce new algorithms for noise mitigation using multi-instance learning. I will cover the experimental results of these models on a dataset made from Freebase.
Diana McCarthy:
Word Sense Models: From static and discrete to dynamic and continuous
(Montag, 12. November 2018)
Traditionally word sense disambiguation models assumed a fixed list of word senses to select from when assigning sense tags to token occurrences in text. This was despite the overwhelming evidence that the meanings of a word depend on the broader contexts (such as time and domain) in which they are spoken or written, and that the boundaries between different meanings are often not clear cut. In this talk I will give an overview of my work, with various collaborators, attempting to address these issues. I will first discuss work to estimate the frequency distributions of word senses from different textual sources then work to detect changes across diachronic corpora. In some of this work we detect such changes with respect to pre-determined sense inventories, while in other work we automatically induce the word senses. One major issue with either approach is that the meanings of a word are often highly related and some words are particularly hard to partition into discrete meanings. I will end the talk with a summary of our work to detect how readily a word can be split into senses and discuss how this might help in producing more realistic models of lexical ambiguity.
Markus Steinbach:
Iconicity in narration. The linguistic meaning of gestures.
(Dienstag, 13. November 2018)
In this talk, I will investigate how sign languages interact with gestures in narration and how iconic gestural aspects of meaning are integrated into the discourse semantic representation of spoken and signed narratives. The analysis will be based on corpus data. In order to account for the complex interaction of gestural and linguistic elements in narration, a modified version of Meir et al.’s (2007) analysis of body as subject and Davidson’s (2015) analysis of role shift in terms of (iconic) demonstration will be developed. One focus will be on quantitative and qualitative differences between sign and spoken languages.
Sven Büchel:
From Sentiment to Emotion: Challenges of a More Fine-Grained Analysis of Affective Language.
(Montag, 26. November 2018)
Early work in sentiment analysis focused almost exclusively on the distinction between positive and negative emotion. However, in recent years, a trend towards more sophisticated representations of human affect, often rooted in psychological theory, has emerged. Complex annotation formats, e.g., inspired by the notion of "basic emotions" or "valence and arousal", allow for increased expressiveness. Yet, they also come with higher annotation costs and lower agreement. Even worse, in the absence of a community-wide consensus, the field currently suffers from a proliferation of competing annotation formats resulting in a shortage of training data for each individual format. In this talk, I will discuss the general trend towards more complex representations of emotion in NLP before reporting on our own work. In particular, we introduced a method to convert between popular annotation formats, thus making incompatible datasets compatible again. Moreover, we achieved close-to-human performance for both sentence- and word-level emotion prediction despite heavy data limitations. I will conclude with two application studies from computational social science and the digital humanities, highlighting the merits of emotion over bi-polar sentiment.
Anders Søgard:
Hegel's Holiday: an Argument for a Less Empiricist NLP?
(Donnerstag, 29. November 2018)
The "empiricist revolution” in NLP began in the early 1990s and effectively weeded out alternatives from mainstream NLP by the early 2000s. These days experiments with synthetic data, formal lanugages, rule-based models, and evaluation on hand-curated benchmarks are generally discouraged, and experiments are based on inducing from and evaluating on finite random samples, rather than in more controlled set-ups. This anti-thesis to early-days NLP has led to impressive achievements such as Google Translate and Siri, but I will argue that there is - not a road block, but - a bottle neck, ahead, a time of diminishing returns. Hegel, however, seems to be on holiday.
Judith Degen:
On the natural distribution of "some" and "or": consequences for theories of scalar implicature
(Dienstag, 18. Dezember 2018)
Theories of scalar implicature have come a long way by building on introspective judgments and, more recently, judgment and processing data from naive participants in controlled experiments, as primary sources of data. Based on such data, common lore has it that scalar implicatures are Generalized Conversational Implicatures (GCI). Increasingly common lore also has it that scalar implicatures incur a processing cost. In this talk I will argue against both of these generalizations. I will do so by taking into account a source of data that has received remarkably little attention: the natural distribution of scalar items. In particular, I will present two large-scale corpus investigations of the occurrence and interpretation of "some" and "or" in corpora of naturally occurring speech. I will show for both "some" and "or" that their associated scalar inferences are much less likely to occur than commonly assumed and that their probability of occurrence is systematically modulated by syntactic, semantic, and pragmatic features of the context in which they occur. For "or" I will further provide evidence from unsupervised clustering techniques that of the many discourse functions "or" can assume, the one that can give rise to scalar inferences is exceedingly rare. I argue that this work calls into question the status of scalar implicature as GCI and provides evidence for constraint-based accounts of pragmatic inference under which listeners combine multiple probabilistic cues to speaker meaning.
Ansprechpartner für Kolloquiumsvorträge
Koordination Kolloquiumsvorträge
- E-Mail schreiben
- Funktionsadresse
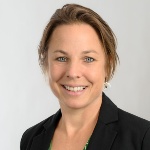
Sabine Schulte im Walde
Prof. Dr.Akademische Rätin